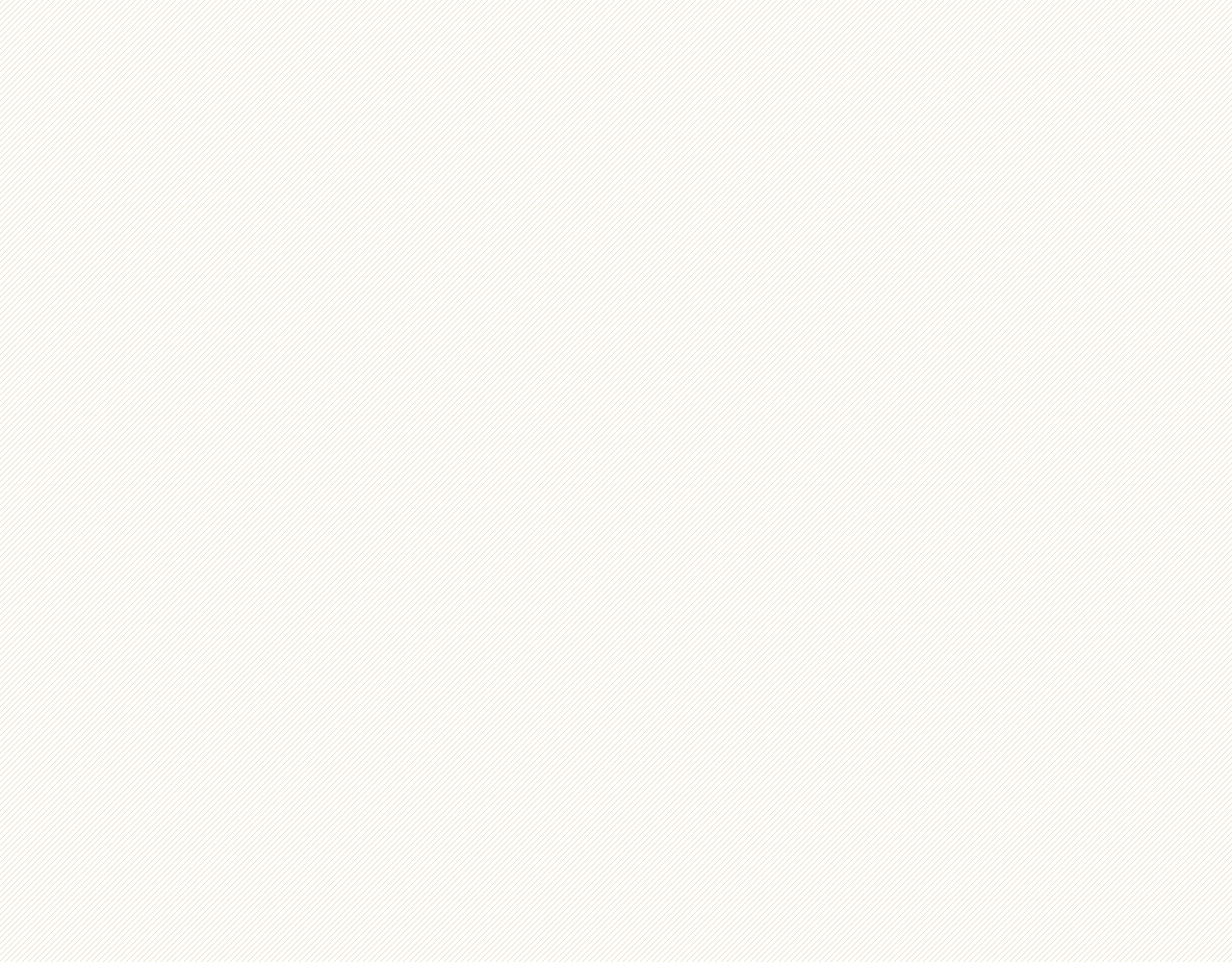






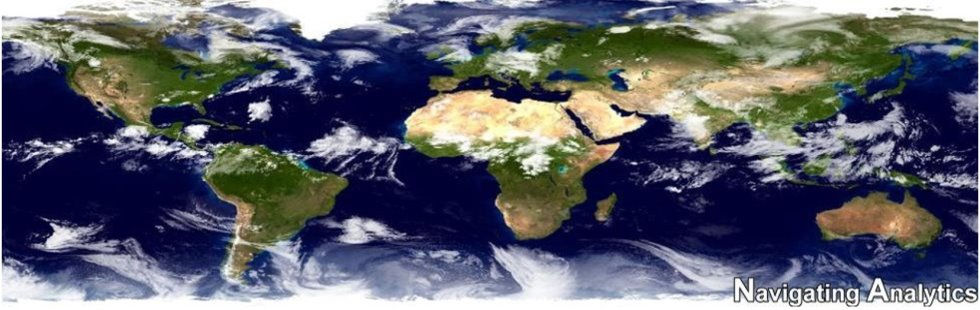
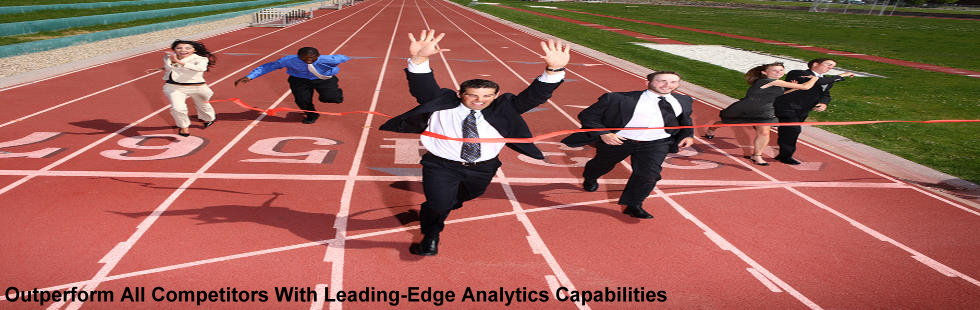
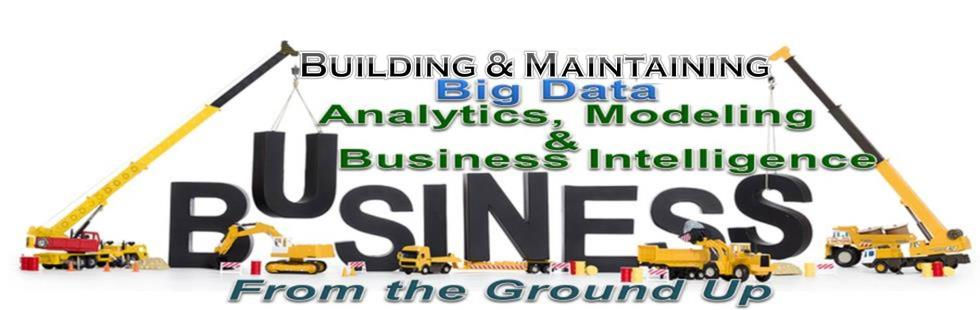
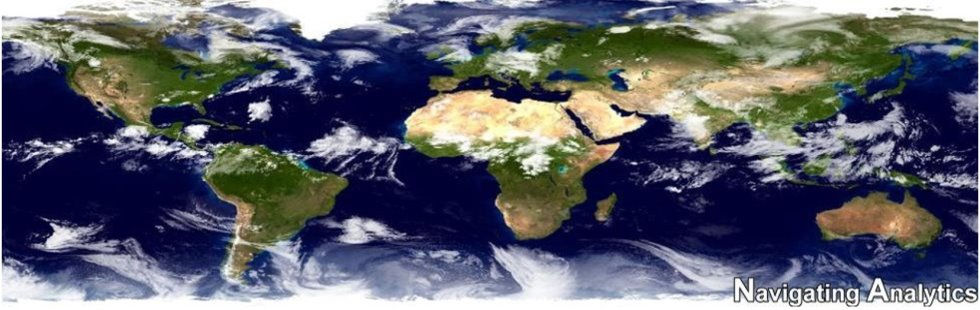
Navigating Analytics
Expertise in Building and Managing Teams, Structures and Processes for Data Management, Analytics, Modeling, Optimization, Business Intelligence Reporting Dashboards and Real-time Streaming from Cloud Technologies

Predictive Analytics: Leveraging the Powerful Enabler to Break Out and Stay Ahead of the Limitations of the Industry
In order for a company to break out of the limitations of its given industry, the business leaders in the company have to manage their businesses of today to become the businesses of tomorrow, next quarter, next year, next three years and next five years of their industry. The leaders have to put together serious long term strategic plans which can be heavily enabled by predictive analytics to drive enormous growth in productivity, product offerings, service offerings, customer acquisitions, customer retention, talent retention, market share, revenue and profitability.
Current Surveys in 2014 and 2015: Recognizing the Importance of Predictive Analytics
In recent years, surveys are showing the increasing use of predictive analytics in many industries.
Leading the industries is the Insurance industry. This is understandable as the adoption of predictive analytics in areas of insurance claims frequency and the severity of these claims are very central to this industry in being able to retain most of the premium and subsequently the profitability for many of the entities. 52 Property and Casualty Insurers participated in the 2014 Predictive Modeling Survey by Towers Watson, the report showed that predictive analytics contributed positively to both the top and bottom lines with increase in rate accuracy (98%), profitability (87%) and market share (41%).
A 2015 marketing survey of 106 B2B marketing executives and CMOs conducted by Forrester Consulting on behalf of Radius on the use Predictive Analytics revealed that the participants were not only exceeding their revenue growth target but also outperforming their contribution percent to the overall revenue. Participants improved their abilities to evaluate new market opportunities (86%), leveraged the insights as the basis for go-to-market strategies (84%), identified new opportunities (89%), analyzed customers in terms of how and why they buy (97%) and enhanced the participants’ abilities to optimize their marketing mix to follow the right buyers (92%).
86 percent of 308 executives who were based in North America confirmed that there were increase in marketing ROI based on the use of predictive analytics in “The Predictive Journey: 2015 Survey on Predictive Marketing Strategies” conducted by Forbes Insights and Lattice Engines.

Does it mean that all the surveys are showing that everything is going well with the use of predictive analytics? Not really. For instance, based on Industrial Internet Report for 2015 put together by Accenture and GE, only 29 percent of the companies surveyed were using Big Data to drive predictive analytics.
In the survey with 308 executives by Forbes Insights and Lattice Engines cited above, only 13% of the companies considered themselves highly advanced with predictive analytics technologies. In the 2015 survey report published by healthdatamanagement.com, only about 15 percent of hospitals providers are using predictive analytics.
As a hands-on predictive analytics practitioner for more than two decades, the conclusion is that there are positive trends in the use of predictive analytics across the industries. This is very impressive and encouraging but there are still very big rooms to leverage predictive analytics for increase in market share, revenue and profitability!
For companies that are trying to break out of the limitations of their industries and stay ahead of their respective industries, here are additional information on predictive analytics that can bring more benefits in terms of increase in market share, productivity, revenue, profitability and of course the actual monetization of predictive analytics as a service, as a product, as a part of an existing business or/and as part of integrated intelligent technologies which are gradually becoming the basis for part of the future internet of things (IOT) processes, products and services.
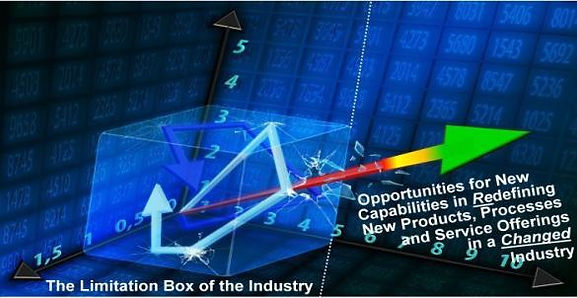
Description of Predictive Analytics
Predictive analytics uses different types of statistical modeling, actuarial modeling, data mining, mathematical modeling, curve fitting, econometrics modeling, structural equation modeling, signal processing, simulation, optimization, neural network and machine learning algorithms to determine the likelihood of an event happening in the future based on the use of historical, processed, inferred, created, simulated, real-time or/and streaming data, behavior and activities.
With integrated algorithms from statistical learning and machine learning, predictive analytics in business environment is an adaptive intelligent layer, with sub layers, that can initiate an action, self-control and based on feedback, self-correct the action if outside the desired ranges, find optimal applicable solutions even within the context of uncertainty and optimize objectives subject to constraints at both dynamic and steady states so as to attain desired performance target for the business while at the same time, it learns the data, searches index, tracks behavior and monitors activities so as to make personalized suggestions and recommendations that help customers find what they are looking for, have positive customer experience, be delighted and have attraction as being part of the business which in the process helps the company to create dopamine digital experiences, increase web traffic, build brand awareness, increase customer retention, influence behavior, drive sales, improve cross-sell, enhance up-sell, lead to increase in order size, improve conversion rate, reduced customer attrition, gain customer loyalty, lower service costs and ensure that the company attains superior and sustainable advantages over the competitors.
Of course, there are pre-requisites that include computing power and prior knowledge of the practitioner.
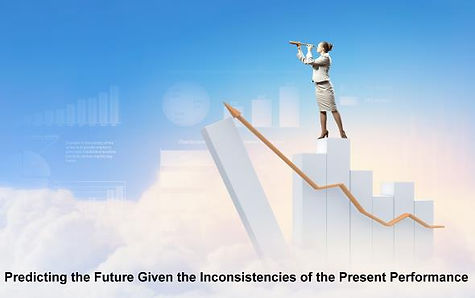
A company that is decisive in breaking out of its limitations can leverage predictive analytics to identify future value-creating products, services, markets, processes, technologies, revenue model, profit model, customers’ behavior and opportunities for alternative course of actions for the business leaders to successfully maneuver in competitive environment. Within the context of strategic planning and leveraging of business capabilities, predictive analytics can be used to predict intention, behavior, outcomes, expected impact, event, consequences, result, cost, benefits and the probability of successful implementation of a given feature of the strategic plan that can result in increase in areas of productivity, customer acquisition, customer retention, market share, revenue and profit that can substantially outperform the industry.
The use of predictive analytics is not limited to business alone as it can predict intention, behavior, outcomes and expected impact in other areas of life that include all industries. The focus of this write-up is narrowly limited to strategic business environment.

There are different techniques in predictive analytics. Predictive modeling is the technique that many people tend to associate with predictive analytics. Some people even think it is synonymous with predictive analytics. This is understandable because many universities across the world teach predictive modeling as part of econometrics, statistics, social sciences, natural sciences and business classes. In addition to predictive modeling, this write-up looks at seven other techniques.
The eight techniques are:
Techniques in Predictive Analytics
-
Predictive Modeling
-
Predictive Profiling
-
Predictive Decisioning
-
Model Predictive Control
-
Predictive Maintenance
-
Predictive Reinforcement and Predictive Representations
-
Predictive Search/ Recommender Systems
-
Predictive Coding
There are still some other prominent and sophisticated predictive analytics techniques like Predictive ADMET, Predictive Toxicology, Predictive Hydrology and Predictive Genetics that are not covered in this write-up. Techniques like Predictive Marketing and Predictive Database Marketing are merged partly with Predictive Modeling and Predictive Profiling. Predictive Index is merged with Predictive Modeling.
Writing about these eight different techniques may seem overwhelming for companies that are trying to make their first entry into the arena where predictive analytics can be used, but this write-up is pointing out that there are additional techniques that can solve other business problems that may have been given up as “unsolvable”. Leveraging partial usage of predictive analytics may produce partial solutions. It can be frustrating for a Call Center leaders that have used predictive modeling to predict the expected numbers of calls, the numbers of agents needed and the average speed of answer (ASA) by seasonality only for the Data Center to have an outage. This is an outage which should have been predicted by predictive maintenance based on the available sensor data.
No matter how good a predictive profiling of the prospective customers may be, if the processes and systems that will handle the increase in the number of customers’ demand are not profiled, then, it is a failure waiting to happen.
In A-Z of Enterprise Analytics, Predictive Analytics is just an integral part of Enterprise Analytics. Others parts of Enterprise Analytics are Strategic Data Management, Operations Analytics, Decision Science Analytics, Digital Analytics, Cloud Based Analytics, Business Intelligence and Prescriptive Analytics that includes Strategic Command Center, Operations Command Center and Customer Value Delivery Optimization Center. A Data Scientist will perform very well in any of these categories depending on the strength and depth of experience in technical and strategic business deliverables.
While the predictive analytics technique that can predict the height of your next born is not included in the eight techniques above, these techniques can easily be used to provide lots of insights that range from using predictive modeling on who to hire to how the company’s revenue stream will look like based on the “hidden” factors causing the variability in the product quality of which Model Predictive Control should have predicted and corrected.
Machine learning is briefly covered towards the end of this write-up because all the predictive analytics techniques have learning algorithms as sub-techniques. Predictive search/recommender systems and predictive coding are heavily dependent on algorithms. These algorithms, even though advanced, are never-the-less, more stable and reduce the time to generate, optimize and automate robust business solutions. Machine learning that are making substantial contributions in predictive analytics include supervised, unsupervised, semi-supervised, reinforcement and evolutionary.
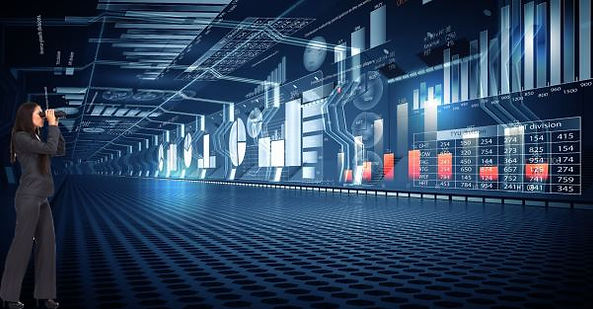
Predictive modeling can provide more information on where your profitable customers are most likely going to go next and what products and services will be attractive to them. It can tell the Operation Teams which work efforts will result in cost reduction and which will generate quicker ROI. It isolates when the next wave of business opportunities is going to show up in your industry and what you need to do while at the same time, it lets you know ahead of time which of your critical employees is looking to leave your company and how that may or may not impact the outcome of your strategic plan.
Predictive modeling can handle the prediction of aggregate demand models, product models, sales models, marketing models, financial models and many more.
Predictive modeling with one of its dimensions in econometrics attempts to leverage economic and business cycle trending data to predict changes in customers’ and business behaviors.
A company that wants to breakout from the limitations of its industry can leverage predictive modeling as the pre cursor of what the market wants, what new products and services to focus on, which geographic areas will be more profitable not only now but in future, what factors can be controlled, which of the factors cannot be controlled and how the uncontrollable factors can be mitigated.
1. Predictive Modeling
Predictive modeling are used in many of the following areas:
-
Strategic Analytics
-
Marketing Analytics
-
Operations Analytics
-
Predictive Modeling Methods for External Data
-
Risk Selection Analytics
-
Premium Risk Analytics
-
Credit Risk Analytics
-
Market Risk Analytics
-
Interest Rate Risk Analytics
-
Exchange Risk Analytics
-
Asset/Liability Matching Analytics
-
Pricing Analytics
-
Liquid Risk Analytics
Predictive Modeling Methods:
Some of the Strategic Analytics Methods
-
Cluster Analysis
-
Conjoint Analysis
-
Principal Components Analysis
-
Correspondence Analysis
-
Fuzzy Classification
-
Ordinary Least Squares (OLS)
-
Generalized Least Squares (GLS)
-
Generalized Linear Models (GLM)
-
Game Theoretical Models
-
Polynomial Regression
-
Partial Least Squares Regression
-
Multidimensional Scaling (MDS)
-
Classification Trees
-
Multivariate Analysis of Variance or Covariance
-
Times Series Forecasting
-
Linear Mixed Models or Residual Maximum Likelihood (REML)
-
Structural Equation Models
Some of the Marketing Analytics Methods
-
Derived Demand
-
Conjoint Analysis
-
Trend and Curve Fittings
-
Discrete Choice Models
-
Variance Analysis Modeling
-
Contribution Analysis Modeling
-
Causal Forecasting Models
-
Projective Forecasting Models
-
Decomposition Analysis Modeling
-
Logit
-
Structural Equation Models
-
Multivariate Probit
-
Design of Experiment
-
Nonlinear Modeling
-
Bayesian Modeling
-
Markov Chain Monte Carlo Methods
-
Hierarchical Linear Models
-
Multinomial Probit Model
-
Stochastic Frontier
-
Principal Components
-
Simultaneous equations modeling
-
Vector autoregressive models
-
ARMA Models: The Box-Jenkins approach
-
Conjoint and Choice Analysis of what the customers want
-
Cluster analysis in market segmentation
-
Adstock model in effectiveness of advertising
-
Multidimensional scaling in customers’ idea points
-
Additional modeling include among others:
-
Modeling Trend and Seasonality
-
Copernican Principle to Predict Duration of Future Sales
-
Leveraging Neural Networks to Forecast Sales
-
-
The Mathematical based behavioral models include among others:
-
Monte Carlo Simulation and sensitivity analysis in terms of Marketing Decision Making
-
Calculating Lifetime Customer Value
-
Efficient Allocation of Resources in Customer Acquisition and Retention
-
Demand Curves with Solver to Optimize Price
-
Conditional probability in bundling and optimization in variance
-
-
Market Basket Analysis and Association Rule
-
OLAP
-
Data Mining See more in Machine Learning
Some of the Operations Analytics Methods
-
Cluster Analysis
-
Conjoint Analysis
-
Principal Components Analysis
-
Correspondence Analysis
-
Fuzzy Classification
-
Causal Modeling
-
Human Capital monitor
-
Network Analysis
-
Decision Trees
-
Frequency Distribution Models
-
Severity Distribution Models
-
Loss distribution approaches
-
Poisson Models
-
Negative Binomial
-
Extreme Value Theory (EVT)
-
-
Neural Networks
-
Design of Experiments
-
Maximum Likelihood Estimation (ML)
-
Moment Estimation Method (MLE)
-
Quantile Estimation Method
-
Generalized Pareto Distributions (GPD)
-
Ordinary Least Squares (OLS)
-
Generalized Least Squares (GLS)
-
Generalized Linear Models (GLM)
-
Game theoretical models
-
Polynomial Regression
-
Partial Least Squares Regression
-
Multidimensional Scaling (MDS)
-
Classification Trees
-
Multivariate Analysis of Variance or Covariance
-
Times Series Forecasting
-
Econometric Modeling
-
Linear Mixed Models or Residual Maximum Likelihood (REML)
-
Structural Equation Models
Modeling External Data that includes Economic Indicators
-
Time Series Analysis
-
ARIMA and ARMAX Models
-
ARCH and GARCH Models
-
Prais-Winsten Regression
-
Vector Autoregression (VAR)
-
Structural Vector Autoregression (SVAR)
-
Vector Error Correction Models (VECM)
-
Nonparametric Time Series Analysis (CAFPE)
-
Smooth Transition Regression (STR)
-
Cointegration
-
-
Simultaneous-Equation Models
Risk Selection Analytics Methods
-
Random Variables and Distributions
-
Expectations and Moments
-
Probability Distributions
-
Maximum Likelihood
-
Claim Frequency and Claim Size Analysis
-
Experience Rating
-
Exposure Rating
-
Bayesian Method
-
Maximum Loss
-
Ordinary Least Squares (OLS)
-
Generalized Least Squares (GLS)
-
Generalized Linear Models (GLM)
-
Polynomial Regression
-
Partial Least Squares Regression
-
Multidimensional scaling (MDS)
-
Classification Trees
-
Multivariate Analysis of Variance or Covariance
Some of the Premium Risk Analytics Methods
-
Probability Distributions
-
Maximum Loss
-
Cluster Analysis
-
Conjoint Analysis
-
Principal Components Analysis
-
Fuzzy Classification
-
Ordinary Least Squares (OLS)
-
Generalized Least Squares (GLS)
-
Generalized Linear Models (GLM)
-
Game theoretical models
-
Polynomial Regression
-
Partial Least Squares Regression
-
Multidimensional Scaling (MDS)
-
Multivariate Analysis of Variance or Covariance
-
Times Series Forecasting
-
Econometric Modeling
-
Linear Mixed Models or Residual Maximum Likelihood (REML)
Some of the Credit Analytics Methods
-
Stress Testing
-
Scenario Analysis
-
Sensitivity Analysis
-
Backtesting
-
Individual Loan
-
Default Risk Models
-
Credit Scoring Models
-
Linear Probability Model (LPM)
-
Logit Model
-
Probit Model
-
Discriminant Model
-
Neural Network (Especially the Probabilistic Neural Network Classifier (PNN) family)
-
Altman Z-score model
-
-
Term Structure Derivation
-
RAROC Models
-
Option Models
-
CreditMetrics
-
Credit Risk+
-
KMV Model
-
Mortality Rate Derivation
-
-
Loan Portfolio
-
Loan Concentration Risk Models
-
Portfolio Diversification
-
KMV Portfolio Manager Models
-
Loan Volume-Based Models
-
Loan Loss ratio models
-
Regulatory models
-
-
Factor Analysis and Factor Loadings
-
Stochastic Risk Measures
-
Mark-to-Market approach
-
Risk-neutral valuation (RNV) approach
-
Extreme Value
-
Maximum loss
Some of the Market Risk Analytics Methods
-
Stress Testing
-
Scenario Analysis
-
Sensitivity Analysis
-
Backtesting
-
Probability distributions
-
Financial Mathematics/ Derivatives Measures. E.g: delta, gamma, rho, vega, theta
-
Mean-Variance
-
Gap Analysis
-
Duration Analysis
-
Capital Asset Pricing Model (CAPM)
-
Modified Form of CAPM
-
Correlations
-
Covariances
-
Copulas
-
Downside Risk Measures
-
Cash Flow at Risk (CFAR)
-
Earnings at Risk (EAR)
-
Capital at Risk
-
Delta-Gamma
-
Parametric VaR
-
Historical Simulation
-
Monte Carlo Simulation
-
Arbitrage Pricing
-
Principal Components Analysis
-
Factor Analysis and Factor Loadings
-
Coherent Risk Measures
-
Option Pricing
-
LIBOR Market Models
-
Approximation Methods
-
Distortion Risk Measures
-
Exponentially Weighted Moving Average (EWMA) Models
-
Generalized Autoregressive Conditional Heteroscedasticity Models (GARCH)
-
Stochastic Risk Measures
-
Market based forecasting
-
Autoregressive Integrated Moving Average (ARIMA)
-
Implied volatility models
-
Exponentially weighted moving average (EWMA)
-
Autoregressive volatility models
-
Autoregressive conditionally heteroscedastic (ARCH) models
-
Generalized ARCH (GARCH) models
-
Fractional integrated GARCH (FIGARCH)
-
Exponential GARCH (EGARCH) model
-
Multivariate GARCH models
-
Asymmetric GARCH models
-
Option Implied Volatility
-
Optimization
-
MCMC (Monte Carlo Markov Chain) approach
-
Extreme Value
-
Maximum Loss
Some of the Interest Rate Risk Analytics Methods
-
Stress Testing
-
Scenario Analysis
-
Sensitivity Analysis
-
-
Backtesting
-
Probability Distributions
-
Financial Mathematics/ Derivatives Measures. E.g delta, gamma, rho, vega, theta
-
Mean-Variance
-
Gap Analysis
-
Duration Analysis
-
Convexity
-
Earnings-at-Risk
-
Capital Asset Pricing Model (CAPM)
-
Correlations
-
Covariances
-
Copulas
-
LIBOR Models
-
Principal Components Analysis
-
Factor Analysis and Factor Loadings
-
Generalized Autoregressive Conditional Heteroscedasticity Models (GARCH)
-
Stochastic Risk Measures
-
Market based forecasting
-
Autoregressive Integrated Moving Average (ARIMA)
-
Simulations
-
Bootstrapping
-
Extreme Value
-
Maximum loss
Some of the Exchange Risk Analytics Methods
-
Market Based Forecasting
-
Autoregressive Integrated Moving Average (ARIMA)
-
Markov Switching Models
-
Threshold autoregressive (TAR) Models
-
Autoregressive volatility (ARV) Models
Some of the Asset/Liability Matching Analytics Methods
-
Stress Testing
-
Scenario Analysis
-
Sensitivity Analysis
-
-
Backtesting
-
Probability Distributions
-
Financial Mathematics/ Derivatives Measures. E.g delta, gamma, rho, vega, theta
-
Mean-Variance
-
Gap Analysis
-
Maturity Model
-
Term Structure
-
Duration Analysis
-
Correlations
-
Covariances
-
Copulas
-
Delta-Gamma
-
Efficient Frontier
-
Principal Components Analysis
-
Factor Analysis and Factor Loadings
-
Coherent Risk Measures
-
Distortion Risk Measures
-
Stochastic programming
-
Decision rules
-
Dynamic stochastic control
-
Multio-bjective linear programming model
-
Goal Programming
-
Exponentially Weighted Moving Average (EWMA) Models
-
Generalized Autoregressive Conditional Heteroscedasticity Models (GARCH)
-
Stochastic Risk Measures
-
Simulation models
-
Portfolio Management Models
-
Arbitrage models
-
Dynamic generalized networks
-
Optimization
-
Extreme Value
-
Maximum loss
Some of the Pricing Risk Analytics Methods
-
Increasing Limit Factor (ILF)
-
Pure Premium
-
Target Loss Ratio Approach
-
Capital-Based Cat Pricing
-
Cluster Analysis
-
Conjoint Analysis
-
Ordinary Least Squares (OLS)
-
Generalized Least Squares (GLS)
-
Generalized Linear Models (GLM)
-
Polynomial Regression
-
Partial Least Squares Regression
-
Multidimensional Scaling (MDS)
-
Classification Trees
-
Multivariate Analysis of Variance or Covariance
Some of the Liquidity Risk Analytics Methods
-
Stress Testing
-
Scenario Analysis
-
Sensitivity Analysis
-
-
Backtesting
-
Liquidity at Risk (LaR)
-
Liquidity Adjusted VaR
-
Exogenous Spread
-
Discount Approach
-
Transaction Cost
-
-
Derivative pricing

Some of the Machine Learning Algorithms Approaches within Predictive Modeling include but are not limited to:
-
Regression Learning Algorithms
-
Regularization Learning Algorithms
-
Nonlinear Regression Learning Algorithms (NLRM)
-
Nonlinear Principal Component Analysis
-
Artificial Neural Network Learning Algorithm
-
Support Vector Machine (SVM) Regression
-
Dimensionality Reduction Algorithms
-
Nonlinear Optimization
-
Kernel methods
-
Clustering Algorithms
-
Association Rules Learning Algorithm
Some of the Regression Learning Algorithms
-
Ordinary Least Squares (OLS)
-
Linear Regression
-
Logistic Regression
-
Stepwise Regression
-
Generalized Least Squares (GLS)
-
Feasible GLS (FGLS) or Estimated GLS (EGLS)
-
Full Estimated GLS (FEGLS)
-
Multivariate Adaptive Regression Splines (MARS)
-
Locally Estimated Scatterplot Smoothing (LOESS)
-
Ordinal
-
Poisson
-
Negative Binomial
-
Log Gamma
-
Quantile
-
Decision Forest
-
Linear Probability Model (LPM)
-
Random Features Regularized Least Squares (RFRLS)
-
Locally Weighted Regression algorithm (LWR)
-
Locally Weighted Projection Regression algorithm (LWPR)
Some of the Regularization Learning Algorithms
-
Least-Squares Method
-
Lasso or Ridge Regression
-
Polynomial Regression
-
Kernel Regression
-
Conditional Random Fields
-
Matrix Decomposition
Some of the Nonlinear Regression Learning Algorithms (NLRM)
-
Log-Linear models
-
Gaussian Mixture Regression (GMR)
Some of the Nonlinear Principal Component Analysis
-
Auto-associative NN for Nonlinear PCA
-
Principal Curves
-
Self-Organizing Maps (SOM)
-
Kernel Principal Component Analysis
-
Nonlinear Complex PCA
-
Nonlinear Singular Spectrum Analysis
Some of the Artificial Neural Network Learning Algorithm
-
Feed-forward neural network models
-
Perceptrons
-
Single-Layer Perceptrons (SLP)
-
Multi-Layer Perceptrons (MLP)
-
-
Back-Propagation Algorithm
-
Hidden Neurons
-
Radial Basis Functions (RBF)
-
Multilayer Perceptron - Artificial Neural Networks (ANNs)
-
Hopfield Network
-
Recurrent Neural Networks
-
Building Self-Organizing Maps (SOM)
-
Radial Basis Function Network (RBFN)
Some of the Support Vector Machine (SVM) Regression
-
Linear SVM Regression
-
Binary and Multiclass Classification
-
Nonlinear SVM Regression
-
SVM Regression Optimization
Some of the Dimensionality Reduction Algorithms
-
Multidimensional Scaling (MDS)
-
Factor Analysis
-
Multivariate Statistical Analysis
-
Principal Component Analysis (PCA)
-
Rotated PCA
-
PCA for Vectors
-
Canonical Correlation Analysis (CCA)
-
-
Nonnegative Matrix Factorization (NMF)
-
Procrustes Function Analyzes
-
Discriminant Analysis
-
Linear
-
Mixture
-
Quadratic
-
Flexible
-
Some of the Nonlinear Optimization
-
Gradient Descent Method
-
Conjugate Gradient Method
-
Quasi-Newton Methods
-
Nonlinear Least Squares Methods
-
Evolutionary Computation and Genetic Algorithms
Some of the Kernel Methods
-
Primal and Dual Solutions for Linear Regression
-
Kernel Ridge Regression
Some of the Clustering Algorithms
Clustering, an unsupervised learning technique, identifies inherent commonality in structures which can be found in set of objects that show similarity measure. Some of the use include vector quantization, features extraction, function approximation, pattern recognition, data mining and image segmentation
Some of the common approaches include:
-
Partitioning Algorithms
-
K-means Algorithms
-
K-medoids Algorithms
-
Density-Based Algorithms
-
Density Functions Clustering
-
Density-Based Connectivity Clustering
-
-
Probabilistic Algorithms
-
-
Grid-Based Algorithms
-
Evolutionary Algorithms
-
Constraint-Based Clustering
-
Co-Occurrence of Categorical Data
-
Hierarchical Clustering Algorithms
-
Agglomerative Algorithms
-
Divisive Algorithms
-
-
Algorithms for High Dimensional Data
-
Scalable Clustering Algorithms
-
Expectation–Maximization (EM) Clustering
-
Support Vector Clustering (SVC)
Some of the Association Rules Learning Algorithm
-
Apriori Algorithm
-
Equivalence Class Transformation (ECLAT)
-
Frequent Pattern growth algorithm (FP-growth)
-
Node-Set-Based Algorithms
-
OPUS Search
-
Multi-Relation Association Rules (MRAR)
-
Context Based Association Rules
-
Generalized Association Rules
-
Sequential Pattern
-
Generalized Association Rules
-
K-optimal Pattern
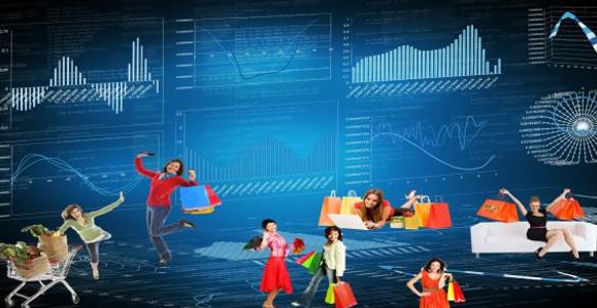
2. Predictive Profiling
Company that wants to go beyond the limitations of the industry can leverage predictive profiling. Predictive profiling includes customer or transaction profiling, competitive profiling, human activity profiling, proactive threat profiling, structural profiling, system profiling, process profiling, market profiling and risk profiling.
Customer or transaction profiling can sense profitable opportunities in the data from demographics, geo-location, age distribution, income, purchasing power, cultural affiliation, education level, behavior, actions, habits, values, attitude, orientation, opinions and buying patterns.
Elements of transaction data that can be captured include the internal relationship between the consumers and the business. It shows the purchasing behavior, the entire purchasing process which includes the product, its categories, the number of items purchased, the size, price of purchase, the type of payment, the method of shipping, the type of discount applied, the state of shipping and the center that handled the initial order.
In profiling the customer, additional data that can provide robust insights include the knowledge of the customers’ data, automated web and SMS behavioral data and data from social media.
Knowledge of the customers’ data includes communication history logs, communication channel preferences, complaint history logs, resolution logs, Net Promoter Score (NPS), previously bought product review, preference for location-based services and life events. Home ownership and credit scores can be of immense value in this category.
Automated web and SMS behavioral data include the customer’s activities with email, mobile messaging, on-web-site behavior that includes abandoned cart, abandoned cart's content and return to abandoned cart etc.
The availability of data from social media can enhance the customer profiling data.
Combining the transaction data, knowledge of the customers’ data, automated web and SMS behavioral data with the social media data provides more holistic and end-to-end information of the customer.
Within the limit of customer privacy, predictive profiling wants to leverage the combined data to build complete picture of the customers with the intention of being able to predict factors that are going to be important for the strategic leadership to use to design, develop and offer products and services that wow the customers.
These combined data can provide robust input into multivariate classification including supervised and unsupervised, CHAID, Regression Trees, Naive Bayes classifiers, logit, Maxent classifier and multilogit algorithm for multiclass classification that can be used in handling customer segmentation while fuzzy classification and predictive database marketing can be used in customer acquisition, customer retention, customer churn, customer engagement, customer lose and win back, customer lifetime value (LTV) and customer satisfaction.
The used of combined data in predictive profiling allows for robust insights that can guide the business leadership in putting together revenue and profitability enhancing strategic solutions in designing and delivering customer centric products and services that the customers value along with well-articulated and operationalized automations that deliver digital solutions which include among others customer engagement strategy as reflected in relevant content, user generated content, usability, consistency and listening post that provide feedback from customers among others.
Predictive profiling goes beyond customer or transaction profiling. As pointed out above, other areas include competitive profiling, human activity profiling, proactive threat profiling, structural profiling, systems profiling, process profiling, market profiling and risk profiling. This is very important to note that a company that wants to implement results from customer or transaction profiling must have insights coming from the profiling of the systems and processes. If a company wants to increase its foot print in a new market, the company must be sure that its systems and processes can handle whatever will be thrown at them with the new additional customers’ demand. And without the competitive profiling, it will be incomplete information for the business leadership to know how the competitors will react or respond. A company that is expanding globally will be smart to include insights from political risk profiling among others.
Predictive Profiling are used in many of the following areas:
-
Customer and Transaction Profiling
-
Statitical and Machine Learning Algorithms cover these areas:
-
Customer acquisition
-
Customer retention
-
Customer churn
-
Customer Lose and win back
-
Customer segmentation
-
Cross-Selling and Up-Selling
-
Multichannel Customer Management
-
Channel Optimization
-
-
-
Competitive Profiling
-
Marketing Profiling
-
Operations Profiling - Especially in the areas of Systems and Processes
-
Risk Profiling
Some of the Customer/Transaction Profiling - Statistical Modeling
-
Conjoint Analysis
-
Cluster Analysis
-
Principal Components Analysis
-
Correspondence Analysis
-
Fuzzy Classification
-
Ordinary Least Squares (OLS)
-
Generalized Least Squares (GLS)
-
Generalized Linear Models (GLM)
-
Game Theoretical Models
-
Polynomial Regression
-
Partial Least Squares Regression
-
Classification Trees
-
Multivariate Analysis of Variance or Covariance
-
Times Series Forecasting
-
Linear Mixed Models or Residual Maximum Likelihood (REML)
-
Structural Equation Models
-
Simultenous Equation Modeling
Some of the Competitive Profiling
-
Conjoint Analysis
-
Principal Components Analysis
-
Correspondence Analysis
-
Fuzzy Classification
-
Ordinary Least Squares (OLS)
-
Generalized Least Squares (GLS)
-
Generalized Linear Models (GLM)
-
Game Theoretical Models
-
Polynomial Regression
-
Partial Least Squares Regression
-
Classification Trees
-
Multivariate Analysis of Variance or Covariance
-
Times Series Forecasting
-
Linear Mixed Models or Residual Maximum Likelihood (REML)
-
Structural Equation Models
Some of the Marketing Profiling
-
Derived demand
-
Conjoint analysis
-
Trend and Curve Fittings
-
Discrete Choice Models
-
Variance Analysis Modeling
-
Contribution Analysis Modeling
-
Causal Forecasting Models
-
Projective Forecasting Models
-
Decomposition Analysis Modeling
-
Logit
-
Structural Equation Models
-
Multivariate Probit
-
Design of Experiment
-
Nonlinear Modeling
-
Bayesian Modeling
-
Markov Chain Monte Carlo Methods
-
Hierarchical Linear Models
-
Multinomial Probit Model
-
Principal Components
-
Conjoint and Choice Analysis of what the customers want
-
Cluster analysis in market segmentation
-
Adstock model in effectiveness of advertising
-
Multidimensional scaling in customers’ idea points
-
Additional modeling include among others:
-
Modeling Trend and Seasonality
-
Copernican Principle to Predict Duration of Future Sales
-
Leveraging Neural Networks to Forecast Sales
-
-
The Mathematical based behavioral models include among others:
-
Monte Carlo Simulation and sensitivity analysis in terms of Marketing Decision Making
-
Calculating Lifetime Customer Value
-
Efficient Allocation of Resources in Customer Acquisition and Retention
-
Demand Curves with Solver to Optimize Price
-
Conditional probability in bundling and optimization in variance
-
-
Market Basket Analysis and Association Rule
-
OLAP
-
Data Mining See more in Machine Learning
Some of the Operations Profiling
-
Conjoint Analysis
-
Cluster Analysis
-
Principal Components Analysis
-
Correspondence Analysis
-
Fuzzy Classification
-
Causal Modeling
-
Human Capital Monitor
-
Network Analysis
-
Decision Trees
-
Frequency Distribution models
-
Severity Distribution models
-
Loss Distribution Approaches
-
Neural Networks
-
Design of Experiments (DOE)
-
Maximum Likelihood Estimation (ML)
-
Moment Estimation Method (MLE)
-
Quantile Estimation Method
-
Poisson Models
-
Negative Binomial
-
Extreme Value Theory (EVT)
-
Generalized Pareto Distributions (GPD)
-
Ordinary Least Squares (OLS)
-
Generalized Least Squares (GLS)
-
Generalized Linear Models (GLM)
-
Game theoretical models
-
Polynomial Regression
-
Partial Least Squares Regression
-
Multidimensional Scaling (MDS)
-
Classification Trees
-
Multivariate Analysis of Variance or Covariance
-
Times Series Forecasting
-
Econometric Modeling
-
Linear Mixed Models or Residual Maximum Likelihood (REML)
-
Structural Equation Models
Risk Profiling
-
Random variables and distributions
-
Stress Testing
-
Scenario Analysis
-
Sensitivity Analysis
-
-
Backtesting
-
Probability Distributions
-
Expectations and Moments
-
Maximum Likelihood
-
Claim Frequency and Claim Size Analysis
-
Experience Rating
-
Exposure Rating
-
Bayesian Method
-
Maximum Loss
-
Ordinary Least Squares (OLS)
-
Generalized Least Squares (GLS)
-
Generalized Linear Models (GLM)
-
Polynomial Regression
-
Partial Least Squares Regression
-
Multidimensional scaling (MDS)
-
Classification Trees
-
Multivariate Analysis of Variance or Covariance

Some of the Machine Learning Algorithms within Predictive Profiling include but are not limited to:
-
Regression Learning Algorithms
-
Clustering Algorithms
-
Association Rules Learning Algorithm
-
Decision Tree Learning Algorithm
-
Control Chart Pattern Recognition Algorithm
-
Ensemble Learning Algorithm
-
Dimensionality Reduction Algorithms
Some of the Regression Learning Algorithms
-
Ordinary Least Squares (OLS)
-
Linear Regression
-
Logistic Regression
-
Stepwise Regression
-
Generalized Least Squares (GLS)
-
Feasible GLS (FGLS) or Estimated GLS (EGLS)
-
Full Estimated GLS (FEGLS)
-
Multivariate Adaptive Regression Splines (MARS)
-
Locally Estimated Scatterplot Smoothing (LOESS)
-
Ordinal
-
Poisson
-
Negative Binomial
-
Log Gamma
-
Quantile
-
Decision Forest
-
Linear Probability Model (LPM)
-
Random Features Regularized Least Squares (RFRLS)
-
Locally Weighted Regression Algorithm (LWR)
-
Locally Weighted Projection Regression Algorithm (LWPR)
Some of the Clustering Algorithms
Clustering is mainly an unsupervised classification learning technique which identifies inherent commonality in structures which can be found in a set of objects which show similarity measure. Some of the use include vector quantization features extraction, function approximation, pattern recognition, data mining and image segmentation
Some of the common approaches include:
-
Partitioning Algorithms
-
K-means Algorithms
-
K-medoids Algorithms
-
Density-Based Algorithms
-
Density Functions Clustering
-
Density-Based Connectivity Clustering
-
-
Probabilistic Algorithms
-
-
Grid-Based Algorithms
-
Evolutionary Algorithms
-
Constraint-Based Clustering
-
Co-Occurrence of Categorical Data
-
Hierarchical Clustering Algorithms
-
Agglomerative Algorithms
-
Divisive Algorithms
-
-
Algorithms for High Dimensional Data
-
Scalable Clustering Algorithms
-
Expectation–Maximization (EM) Clustering
-
Support Vector Clustering (SVC)
Some of the Association Rules Learning Algorithm
-
Apriori Algorithm
-
Equivalence Class Transformation (ECLAT)
-
Frequent Pattern growth algorithm (FP-growth)
-
Node-Set-Based Algorithms
-
OPUS Search
-
Multi-Relation Association Rules (MRAR)
-
Context Based Association Rules
-
Generalized Association Rules
-
Sequential Pattern
-
Generalized Association Rules
-
K-optimal Pattern
Some of the Decision Tree Learning Algorithm
-
ID3 (Iterative Dichotomiser 3 - Ross Quinlan)
-
C4.5 and C5.0 algorithms - Successor of ID3
-
Classification and Regression Tree (CART)
-
Chi-squared Automatic Interaction Detection (CHAID)
-
Conditional Decision Trees
-
Decision Stump
-
M5
-
MARS (Multivariate Adaptive Regression Splines) Algorithms
Some of the Control Chart Pattern Recognition Algorithm
-
Learning Vector Quantization (LVQ) Network
-
Multi-Layer Perceptrons (MLP)
-
Generalized Learning Vector Quantization
Some of the Ensemble Learning Algorithm
-
Bayes Optimal Classifier
-
Bootstrap Aggregating (Bagging)
-
Random Forest
-
AdaBoost
-
Bayesian Parameter Averaging (BPA)
-
Gradient Boosting Machines (GBM)
-
Bayesian Model Combination (BMC)
-
Boosting
-
Randomized Trees
-
Random Subspace
Some of the Dimensionality Reduction Algorithms
-
Multidimensional scaling (MDS)
-
Factor analysis
-
Multivariate Statistical Analysis
-
Principal component analysis (PCA)
-
Rotated PCA
-
PCA for Vectors
-
Canonical Correlation Analysis (CCA)
-
-
Nonnegative Matrix Factorization (NMF)
-
Procrustes Function Analyzes
-
Discriminant Analysis
-
Linear
-
Mixture
-
Quadratic
-
Flexible
-
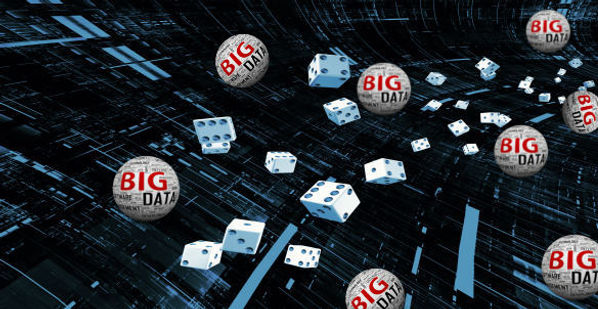
3. Predictive Decisioning
There are times that a company’s leaders may not have access to all the information that they need. Predictive decisioning presents an opportunity. This technique focuses on predicting under uncertainty. Making business decisions requires the business leaders to reach coherent, credible and probabilistic conclusions in the midst of large set of data that are not only noisy, with disjointed information and at times, dealing with illogical factors coming from economic, social, political, technological environments and in fact from the consumers’ and employees’ behaviors. There are situations when predictive modeling may not be sufficient to provide the necessary insights that the business leaders need to make decisions.
Subject to different types of constraints, predictive decisioning framework becomes the central approach as the framework allows for leveraging various sources of uncertainties to be integrated so that all types of variability can be captured.
Unlike predictive modeling, this framework allows for the addition of model parameters until the optimal inference and prediction quality is attained.
Prediction decisioning or technically called Bayesian starts with a very simple model. There is the increase in the level of model flexibility and uncertainty while at the same time solving for the given problem. This process continues in increasing complexity with additional uncertainties until there is broad inclusion of all uncertainties as reflected in the model parameters and the constraints with eventual optimal output. Predictive decisioning works through making sure that adding additional factors does not increase the error in the model. Also there is the question of improving the model by looking at additional factors on how it improves the likelihoods and take care of other technical tests.
Predictive decisioning is very adaptive within the context of uncertainty at seeking to maximize information available to the decision makers. This involves the focus on finding the optimal inference to maximize the expected utility including the quality of the decisions. The predictive decisioning is very important in figuring out choice models, demand models and consumer preferences models. The business leaders have the responsibility to simultaneously estimate demand and supply to maximize profitability within the context of uncertainty.
Types of Learning in Predictive Decisioning:
-
Probabilistic Models and Curve Fitting Learning
-
Inference Learning
-
Statistics for Learning
-
Bayesian Learning Algorithm
Some of the Probabilistic Models and Curve Fitting Learning
-
Beta Distribution
-
Double Exponential Distribution
-
Exponential Distribution
-
Gamma Distribution
-
Generalized Gamma Distribution
-
Normal Distribution
-
Pareto Distribution
-
Uniform Distribution
-
Weibull Distribution
-
Log-Normal Distribution
-
Uniform Distribution
-
Gamma Distribution
-
Inverse Gamma distribution
-
Laplace Distribution
-
Univariate Gaussian Distribution
-
Student’s t-distribution
-
Dirichlet Distribution
-
Multivariate Gaussian Distribution
-
Discrete Distribution
-
Bernoulli Distribution
-
Beta-Binomial Distribution
-
Binomial Distribution
-
Negative Binomial Distribution
-
Poisson Distribution
-
-
Multivariate Distribution
-
Dirichlet Distribution
-
Wishart Distribution
-
Student’s t Distribution
-
Multinomial Distribution
-
Some of the Inference Learning
-
Naive Bayes
-
Gaussian Naive Bayes
-
Multiclass Naive Bayes
-
-
Bayesian Belief Network (BBN)
-
Bayesian Network (BN)
-
Hierarchical Model
-
Generalized Linear Mixed Model (GLMM)
-
Logit GLMM
-
Poisson log-linear GLMM
-
-
-
Single-Parameter Models
-
Multi-Parameter Models
-
Multinomial Model
-
-
Genetic Linkage Model
-
Generalized Logit Model
-
Variance Components Model
-
Mixture of Exponentials Model
-
Hidden Markov Model (HMM)
-
Markov Chain Monte Carlo Methods (MCMC)
-
Discrete Markov Chains
-
Metropolis-Hastings Algorithms
-
Gibbs Sampling
-
Component-wise Metropolis-Hastings
-
Metropolis within Gibbs
-
Some of the Statistics for Learning
-
Dynamic Linear Model (DLM) or Kalman Filter setup
-
Bayes’ Estimation
-
Multiple Regression
-
Time series DLM
-
Polynomial Trend Models
-
polynomial Growth Models
-
Seasonal Models
-
Form-Free Seasonal Effects DLM
-
Fourier Form Seasonal Effects DLM
-
Reduced Fourier Form Models
-
-
-
-
Non-Linear Dynamic Models
-
Exponential Dynamic Models
-
Generalized Linear Models
-
-
Multivariate Modeling and Forecasting
-
Hierarchical and Panel Data Models
-
Times Series
-
Autoregressive Moving Average Models (ARMA models)
-
Time Series Decompositions
-
Time-Varying Autoregressive
-
Stochastic Models
-
ARCH and GARCH Models
-
Volatility Models
-
-
-
Bayesian Linear Regression
-
Univariate Linear Regression Model
-
Multivariate Linear Regression Model
-
-
Polytomous and Ordinal Regression
-
Robust Regression
-
Hidden Markov Model (HMM)
-
Spatial Regression
-
Spatio-Temporal Models
-
-
Structural Equation and Latent Variables
-
Models for Classification
-
Naive Bayes Classifier
-
Discriminant Functions
-
Least Squares Approach
-
Fisher’s Linear Discriminant
-
Perceptron Algorithm
-
-
Probabilistic Generative Models
-
Probabilistic Discriminative Models
-
Generalized Linear Model (GLM)
-
Logistic Regression
-
Probit Regression
-
Negative Binomial Model
-
Generalized Poisson Model
-
Inflated Models
-
Bivariate Poisson Model
-
Poisson Difference Model
-
Multinomial Logistic Regression
-
Bayesian Logistic Regression
-
-
Deterministic Methods
-
Laplace Approximation
-
Expectation Propagation Algorithm
-
Variational Gaussian Approximation
-
-
Simulation
-
Independent Simulation
-
Rejection Sampling
-
Importance Sampling
-
-
State-Space Autoregression
-
Dependent Simulation
-
MCMC Methods
-
Metropolis–Hastings Algorithm
-
Gibbs Algorithm
-
Hybrid MCMC Methods
-
-
-
Transition Mixtures
-
Metropolis within Gibbs
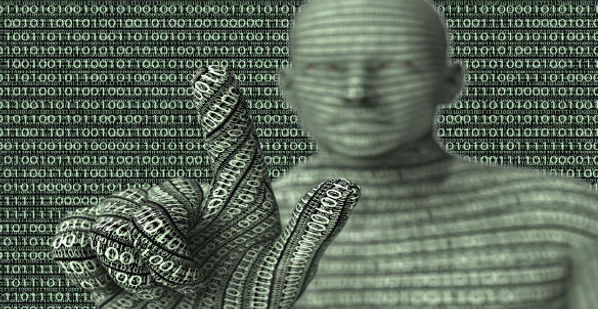
Some of the Bayesian Learning Algorithm
-
Naive Bayes Optimal Classifier
-
Gaussian Naive Bayes
-
Brute-Force Maximum a Posteriori (MAP) Hypotheses and Consistent Learners
-
Type-II Maximum Likelihood
-
Markov Chain Monte Carlo (MCMC)
-
Averaged One-Dependence Estimators (AODE)
-
Expectation Propagation (EP)
-
Laplace Approximation
-
Bayesian Belief Network (BBN)
-
Variational Approximations
-
Multinomial Naive Bayes
-
Bayesian Information Criterion (BIC)
-
Bayesian Network (BN)
-
Exact Sampling
-
Gaussian mixture model (GMM)
-
Generative Model
-
Latent Dirichlet allocation (LDA)
-
Discriminative Model
Some of the Nonlinear Classification
-
Multi-Layer Perceptron Classifier
-
Multi-Class Classification
-
Bayesian Neural Network (BNN) Classifier
-
Support Vector Machine (SVM) Classifier
-
Forecast Verification
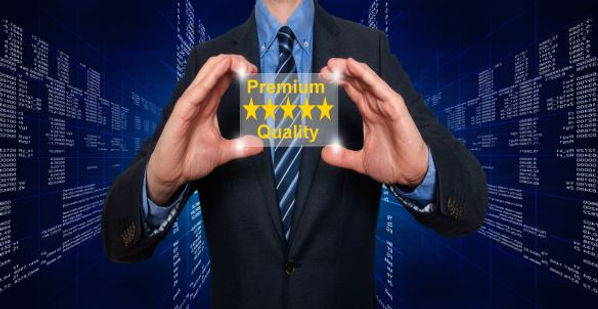
4. Model Predictive Control (MPC)
Model Predictive Control (MPC) is the predictive analytics family member that can start your car and it can stop your car when it senses danger or when it detects that there is collision about to happen. It can tell you to take a break from your driving because the signal from your seat is sending information that your body mass is not active enough. It is one of the unseen “co-driver” driving the car with active steering, adaptive cruise control and traction control.
MPC helps with satellite altitude control, shows up as one of the unseen crew of unmanned aerial vehicles and for a manufacturing company trying to perform better than the industry, MPC holds the insights on how to reduce cost, ensure consistency of product quality, reduce emission level and generate sustainable profit.
For companies that are in financial markets, MPC can handle different types of linear, constrained linear, stochastic, and dynamical portfolio optimization in financial engineering.
Some of the Machine Learning Algorithms Approaches focus on multi-input multi-output processes with:
-
Neural Networks
-
Double-Layer Perceptron
-
Locally Recurrent Globally Feedforward (LRGF)
-
Hammerstein and Wiener Models
-
Neural State-Space Models
-
Neural Multi-Models
-
Neural Approximation
-
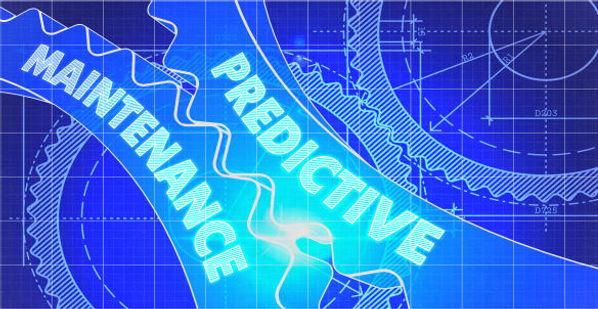
5. Predictive Maintenance
Predictive Maintenance predicts the failure of physical assets. It can also predict the point of failure of a component or part of the physical asset so that the component or the part can be replaced. This predictive analytics technique can ground a commercial jet because it can predict how big the tiniest crack in a structural component will be by the next airport landing. 53 customers with over 2,000 aircraft depend on Boeing's Airplane Health Management (AHM) and 106 customers of Airbus are with Aircraft Maintenance Analysis (Airman).
Predictive Maintenance can help a business gain competitive differentiators to break out and stay ahead of the industry. There are many Call Centers across the nation on daily basis that have everything ready to go until there is Data Center outage. Based on the findings from Ponemon Institute and Emerson Network Power on 67 Data Centers located throughout the nation, the 2013 full economic cost of unplanned data center outages ranged from $74,223 to $1,734,433 per organization with an average cost of $627,418 per incident. According to Gartner, in 2014, network downtime cost $5,600 per minute which translated to over $300K per hour.
For a company, the business goals based on the insights from predictive modeling and predictive profiling can easily be missed if there is a major problem with the structural component in the business building site. This is where predictive maintenance steps in to make sure that the health of the building site and other physical assets are part of the predictive information for actionable insights.
Predictive maintenance is very important to business leaders especially those in operations, manufacturing, production and maintenance in asset-intensive industries because the success of their strategic plans may have to do with the health of the company’s physical assets and the components. Whether the strategic plan will maximize revenue, minimize expense or/and generate operating profits, may depend on the health of the physical assets.
The asset-intensive industries include manufacturing, processing, mining, public utilities, transportation, defense, aerospace, oil and wind power.
In the past, corrective maintenance and preventive maintenance were prevalent. Corrective maintenance involves restoring or repairing an asset to a working or operational condition after failure has happened. In what is called “run-to-failure”, many business leaders operate on the principle that if it is not broken, there is no need to fix it.
Preventive maintenance is based on planned maintenance that is driven by meters, timeline related and generating events. Many of these planned maintenance is based on the manufacturers’ recommendations of when the parts are to be removed, readjusted, serviced or replaced. Preventive maintenance entails series of tests, measurements and monitoring. Monitoring statistics include Mean-Time-Between-Failure (MTBF) or bathtub Curve. The maintenance schedule is determined by a prescribed timeline. There are no serious attempts to integrate the information on how the physical asset is used, when it is used, where it is used and if it is being used differently as opposed to how it was recommended.
Preventive maintenance over time has not been able to:
-
Prevent emergency due to breakdown of equipment
-
Reduce the cost of maintenance including the cost of parts and repair costs
-
Ensure stability in workforce management
-
Prevent high wait time whenever there is a breakdown in the equipment
Predictive Maintenance is one of the family members of predictive analytics that can help a business gain competitive differentiators as there is the prediction of the health of the company’s physical assets.
Predictive maintenance predicts failure before it actually happens by directly monitoring the machine condition, efficiency and other parameters during normal operating condition and sending the information to where they can be monitored or detected, analyzed and corrected.
Some of the areas monitored include relative and absolute vibration, distance for the Shaft sensor, load condition and rotation axial positions.
There are different types of sensor information that are collected. The signal data can be static, semi-static, harmonic, modulated, pulsed, random, dynamic and processed. Static data includes load condition, vibration levels and bearing temperature. Semi-static includes shaft position. Harmonic data covers misalignment; modulated data embodies eccentricity and pulsed data includes surge, random data includes lubrication issues. Dynamic data includes vibration and rotation signals and processed data includes signals which are measured as function of time or time series.
The sources of data include maintenance records, historical records which cover inspection and test records, standard maintenance schedules information and real-time streaming signal sensor data.
In paying attention to predictive maintenance, business leaders have insights that include:
-
When and where there are most likely going to be failure
-
What will probably be the cause of the failure
-
Based on integrated root-cause analytics, why will there be a failure
-
How to efficiently and effectively manage production capacity
-
How to improve safety control, ensure compliance and manage risk mitigation program
Predictive Maintenance algorithms can generate insights that provide the business leadership with more control in terms of:
-
Being able to keep running the machines that are essential to meet customers’ order and ensure customer satisfaction, customer retention and generate revenue
-
Reduction in the cost base and total cost of maintenance
-
Avoidance of unnecessary equipment breakdown, minimize possibility of down time and reduce part inspection frequency that can all combine or individually prevent the business from achieving its goal
-
Have a predictive workforce management in terms of being able to stabilized workforce, workloads and ensure productivity.
Some of the Machine Learning Algorithms Approaches focus on:
-
Neural Networks which includes
-
Multilayer Perception (MLP)
-
Feedforward Networks
-
Recurrent Networks
-
Kohonen network or self-organising map
-
-
Support Vector Machines (SVMs)
-
Support Vector Regression
-
Support Vector Classification
-
-
Classification Using Genetic Algorithms
-
Unsupervised Learning
-
Nonparametric Density Estimation
-
Non-Gaussian Normal
-
Gaussian-Distributed Normal
-

This predictive technique focuses on where an autonomous agent will attempt to maximize the amount of reward that it can receive by associating optimal behavior with reward signals base on the past actions and continuation of the actions. Predictive reinforcement is designed to derive closed-loop policies for stochastic optimal control problems based on the collection of information gathered from the agent or gathered from simulations. It simply shows that an agent will follow reasonable and effective policy in a given state so as to get the maximum expected result.
This technique can be used from robotic navigation to operations research in the areas of scheduling and warehousing.
The strength of the prediction is in its ability to capture as much of the regularities that enhance the ability of the agent to learn as quickly as possible so as to accumulate more rewards. Companies have opportunities to use this approach in terms of understanding the compensation and benefits packages that are given to the employees and the consequences on productivity, revenue and profitability.
6. Predictive Reinforcement and Predictive Representations
Some of the Machine Learning Approaches focuses on:
-
Regression Learning Algorithms
-
Clustering Algorithms
-
Association Rules Learning Algorithm
-
Decision Tree Learning Algorithm
-
Ensemble Learning Algorithm
-
Dimensionality Reduction Algorithms

7. Predictive Search Algorithms or the Recommender Systems
When searching on Google, Bing, Yahoo, Yandex, Baidu, Sogou, T-Online, Conduit or any of the major search engines, the underlining drivers of the suggestions or autocomplete that show up are based on predictive search algorithms.
In 2015, even though the tech driven Nasdaq rose by 5.7 percent, the Dow was 2.2 percent under and so also the S&P was under by 0.7 percent but there were four stocks that had an average returns of 83 percent between them.
The 2015 stock market would have been toothless except that there was FANG! FANG stands for Facebook, Amazon, Netflix and Google (Alphabet). Facebook’s performance was at 37 percent, Amazon came in at 118 percent, Netflix was at 134 percent and Google (Alphabet) delivered at 47 percent.
What these four companies have in common are predictive search algorithms or the recommender systems. Facebook's "People You May Know" Amazon’s “Customers Who Bought This Item Also Bought” Netflix’s Recommendation “Because you watch __” and “Other movies you might enjoy” and “Google Suggest” or Google “Autocomplete” or Google “Knowledge Graph” or “Google Now”.
The algorithms suggest the words, phrases or related words and related phrases. In addition, these algorithms suggest and recommend products and services that are related to the products and services that customers are reviewing or looking to purchase.
This technique anticipates the next clicks, makes recommendations, identifies what other products that are related to what is being viewed, allows for comparison of the products, attaches satisfactory “stars”, provides links to feedback of previous buyers and reviewers and in some cases, provides links to the manufacturers and to other sellers that may have related products and services and encourages the customers to purchase more of the same products and services.
While this write-up does not believe there is a correlation between the stock performance and the predictive search algorithms or the recommender systems, what is obvious is that this predictive technique is very central to how these companies are using predictive algorithms to reach out to their customers at the point of closing the sale. According to Amazon, 35 percent of its sales came from the recommenders. Google generates 38 percent additional click-throughs from recommendations and 66 percent of movies watched in Netflix were based on the recommenders.
The predictive search or the recommender systems come with different sub-techniques. These include context-aware, content-based and collaborative filtering among others. The context-aware recommender is a combination of factors of state that the user was at the time of buying or using. Some of these factors can include time, geo-location position and event. Content-based recommender use past behavior to predict similar item that customer had bought or used. Collaborative filtering uses rating information from nearest neighbors or other users of the item to recommend to the new user if the users and the new user have rated the same item the same way. Some of the approaches for collaborative filtering include model based and memory-based.
Model based collaborative filtering algorithms are learned from the data and can take the form of matrix factorization, association rules, clustering and Restricted Boltzmann machines, a generative stochastic form of Neural Network. The memory based collaborative filtering include the predictive rating for the item and predictive ratings for the target user's item.
There are different types of social recommenders like extended collaborative filtering which is driven by social neighborhood and link prediction algorithms that are being used by social network platform providers where existing users are recommended to the new user.
In addition to the algorithms above, there are other algorithms that include:
-
Context-aware
-
Content-based
-
Collaborative Filtering
-
“Friend-of-Friend” (FoF) Algorithm
-
Fagin’s Threshold Algorithm
-
Inverted Index Approaches
-
Predictive Indexing
-
Latent Semantic Indexing
-
Halted threshold Algorithm
-
Polynomial Semantic Indexing
-
Probabilistic Latent Semantic Indexing
Apple’s Siri and Microsoft Cortana’s Virtual Assistant are based on new generation of these algorithms.
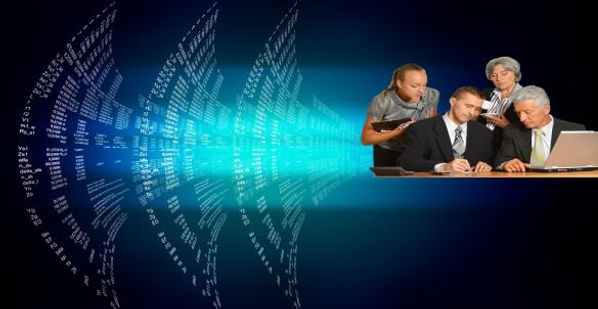
8. Predictive Coding
Predictive coding takes care of making available relevant documents as part of electronically stored information (ESI) for regulatory audit and litigation requests in e-discovery as well as being able enhance the absorptive capacity of a company.
In regulatory audit and litigation requests, predictive coding can go through millions of documents in order to find relevant Information during e-discovery. Predictive coding was endorsed by the Judicial system in Da Silva Moore v. Publicis Groupe (S.D.N.Y Apr. 26, 2012). In Global Aerospace, Inc., et al. v. Landow Aviation, LP, et al, the Judge affirmed the use of predictive coding to review about two million documents in what could have caused 20,000 man-hours or two million dollars.
Companies that are determined to exceed the performance of their peers with acquisition of absorptive capacity will find predictive coding very useful. In combining the external information with internal information that are needed for competitive edge, predictive coding provides machine learning algorithms that make accessibility of information easier to the strategic employees that need to make decisions that can be decisive for the company’s revenue and profitability.
Some of the Algorithms include:
-
Latent Semantic Indexing (LSI) which is a variant of decision trees
-
Support Vector Machines (SVMs)
-
Naïve Bayes Classifier
-
Probabilistic Latent Semantic Analysis

Machine Learning Algorithms in Predictive Analytics
The intersection of computer science, mathematics, operations research, econometrics, statistics and a sub branch of artificial intelligence or AI is creating machine learning algorithms that are taking predictive analytics to the next level. Machine Learning algorithms are finding welcoming and increasing applicability in predictive analytics.
The machine learning algorithms can generate and automate robust solutions that smart company can take and use not only for tactical efficiency and productivity but also for sustainable long term business growth.
While econometricians and statisticians are concerned about modeling the data, the machine learning is focusing on learning from the data. It is a little concerning for those who argue that if the data is bad, the learning parameters, models and approximations may be compromised. The issue of data quality is not going to be solved easily but then, there are ways to mitigate the issue. The traditional data cleansing process will continue to help and that is why it is very important to have an expert that understands the company's data.
Also, algorithms that integrate data profiling can be developed to show where the quality of the data may be an issue. In future, data quality safe-guarding algorithms can be integrated to prevent the lowering of the data quality going forward after the first cleansing exercise.
As earlier pointed out, the machine learning includes supervised, unsupervised, semi-supervised, reinforcement and evolutionary.
Supervised Learning: The approach learns the data to discern or discover relationship between the target attribute or the dependent factor and the input attributes by using a teaching signal or desired output signal. The essence of the learning is to discover the hidden relationship and use it to explain and predict the target value. There are two prominent types of supervised learning approach – Regression models and Classification Models or Classifiers. The Regression modeling which is used on continuous factors will predict real value while the Classifiers which is used on discrete factors classify into predefined groups. Unlike the regression modeling in econometrics and statistical modeling where the focus is on prediction, supervised learning includes both the prediction and the optimization because by its nature, it looks for optimal value as in regression models or optimal grouping as in classification models. Supervised learning can be applied to off-line and on-line labeled data. There are three sets of data that include training set, testing set and validation set. There are times we may also have the cross validation set to ensure that the dataset from the original dataset has the same chance of appearing in the training set and testing set.
Unsupervised Learning: The unsupervised learning works on unlabeled data and it seeks to find hidden relationship. Unsupervised learning, more of ad hoc, learns data points which have no labels through algorithms and can be used to remove anomaly, reduce data dimensionality, group data based on similarity and estimate probability distribution. Manifold learning is a variant of this especially where there are two or more dimensions in the data and there is non-linear structure within the data. Manifold learning allows for learning of the multi dimensionality from the data itself so as to escape from the “curse of dimensionality”.
Semi-supervised Learning: This involves learning from both labeled and unlabeled data which means learning from the input from the teacher and learning based on similarity.
Reinforcement Learning: Rewards and payoffs are the basis of running risk mitigation environments. The questions to ask include: Are there opportunities of reinforcing the factors that enhance the mitigation through ensuring the continuation of the reward? So if the past works, in a Markov process modeling, why not reinforce with reward to keep it going? What then becomes the type of reward and how best to implement the reward tend to combine the learning methods with strategic implementation which generate the structures and processes for sustaining the needed behavior.
Evolutionary Learning: Can we use the natural evolution as the basis for computational intelligence that enhances predictive analytics? The answer is yes! Evolutionary learning or Evolutionary algorithms (EA) is using bio-inspired to leverage, fitness, initialization, selection, mutation, reproduction and variation as the basis for stepwise in learning, adaptation and optimization. Prominent algorithms within the EA are the Genetic algorithms (GA), Classifier systems (CS), Messy genetic algorithms, Evolution strategies (ESs), Cultural algorithms and the Swarm intelligence based algorithms which include among others Fish swarm algorithm, Ant colony optimization, Bee colony algorithm, Artificial bee colony, Artificial immune systems, Bat algorithm, Cuckoo search, Firefly algorithm, Gravitational search algorithm, Flower pollination algorithm, River formation dynamics, Multi-swarm optimization, Gray wolf optimization and Wolf search algorithm.
A company ready to leave the competitors in the dust can benefit from the fact that predictive analytics including machine learning algorithms is a competitive differentiator as it can provide profitable insights in areas that include customer-centric and customer facing strategy, customer strategy development, customer portfolio strategy, customer segmentation, channel strategy development, customer management, customer relationship management, customer communications management, channel management, channel optimization, customer operations, customer identity management, customer communications, service level management and customer lifetime value (LTV).

Current Factors That Are Strengthening Predictive Analytics
Shortening are the days when predictive analytics used to struggle to get a good model in the predictive modeling department. Today, there are lots of factors that are coming together at the same time to strengthen and drive the internal consistency, reliability and computational applicability power of predictive analytics to new levels.
An important factor is really about the “past data” which requires paging Large Hadron Collider (LHC) on Higgs Boson Factory at CERN. There may be those who view predictive analytics as using the past data to predict the future occurrence. That view will have to be relegated to yesterday. Why? Today, thanks to the advancement in technologies and the use of advanced algorithms, it is easier to go beyond relying on the past data alone.
The past data can now be expanded in dimension to include the current real-time streaming data. Also in addition, the past data can be algorithmic represented by interpolating the intended decision of now and see how much it could have changed the past data. This is not back-testing or retrodiction. This is contextualizing now as if it has happened or happening now!
Built by Conseil Européen pour la Recherche Nucléaire (CERN), Switzerland, the Large Hadron Collider (LHC) is the world's largest data producer and in May 2015, it successfully delivered high-power collisions of sub-atomic particles at 13 teraelectronvolts (TeV). Part of the deliverables for LHC include the predictions of different theories of particle physics based on the data that it is creating now!
This means that the definition of what is "past data" can now be contextualized by the experience of the individual or team working with the past data. We are now going beyond the limitations (including "past data") of what had prevented us in predictive analytics from being able to provide more robust predictive information to enhance the trajectories of our respective companies in their industries.
Other factors that are strengthening and driving the power of predictive analytics to new levels include the availability of big data with volume, variety, velocity and veracity that can be leveraged; the Hadoop ecosystem which integrates open-source data management technologies, programming tools, application programming interfaces (APIs) and other analytics friendly utilities for reliable, scalable, flexible, fault-tolerant distributed computing with the capacity to abstracts many complexities in distributed and concurrent multiple applications; the Massively Parallel Processing (MPP) which overcomes many of the limitations of uni-processor and Symmetric Multi-Processing (SMP); the In-Memory Distributed Data Grids which has the ability to leverage horizontal scalable approach to support existing database to provide super-fast data access to application; the horizontal scalable No-SQL with its highly flexible model dependent on the emerging dimensionality of the data which can provide additional pathways to predictive analytics practitioners and modelers to leverage schema free, non-relational, semi-structured and unstructured; the availability of Data Stream Management System which provides for continuous, real-time data stream that allows for analytics of rapidly changing data; the Event Processing which allows for the continuous ingestion of new set of event data that can help the Predictive Analytics Team to detect not only the strength and relevance of the existing models or the scoring models but can also be a source of validation of the quality of service requirements that should exist between the business and the predictive analytics leaderships.
Additional factors also include the In-Memory Databases that provide the quick access in seek time, reliability and predictability needed to test and validate business strategies of the future and the lessons learnt from Large Hadron Collider (LHC) on new ways to be able to analyze gigabytes of data by leveraging Grid Computing technologies which allow for connecting computing centers that can be used to handle data rich complex predictive analytics.
The Cloud-Based Solutions provide for data storage, modeling and scoring models which can be created, validated, deployed, monitored, managed and updated if and when needed plus the on-demand business intelligence delivery.
And finally, the coming together of econometrics, statistics, mathematics, operations research, computer science and a sub branch of artificial intelligence or AI which is producing powerful Machine Learning algorithms.
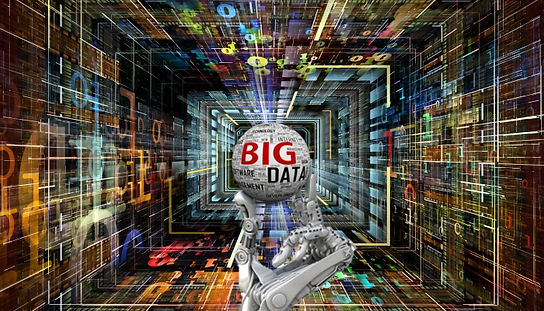
The Outlook for Predictive Analytics is Even More Promising
The future prospects of Predictive Analytics are in fact more promising. There are many factors in future that will enhance the power of predictive analytics. These factors include opportunities to increase the processing power given the fact that we are going to generate more data from current and new sources. The advent of fast computing power like China’s supercomputer Tianhe-2 (MilkyWay-2), the fastest computer in the world, is being matched by the US President’s order of July 29th, 2015 to build the exascale computers which will overtake China’s supercomputer Tianhe-2. The increasing processing power should accelerate business deliverables.
The emergence of Quantum Computing with qubits that go beyond treating data as binary digits (0’s or 1’s) is displaying unlimited power to handle extreme rapid, complex, streaming and refactoring calculations with thousands of interacting categorical and continuous variables. Quantum computing will allow predictive analytics to leverage two of its features which include entanglement and superposition. Statistically, in analyzing independent variables in modeling, we in analytics “throw” away independent variables as “insignificant”.
The entanglement nature in quantum computing can potentially be used to process the correlated part of the “insignificant” independent variables so as to increase the coefficient of determination, the R-squared or percent of variance explained of the dependent variable. The superposition principle becomes the precursor for addition of two or more-time series. This is will help in generating robust predictive modeling for business and stock market performances!
With entanglement and superposition, quantum computing can process vast number of calculations simultaneously. Classical computer works with ones and zeros, a quantum computer has the advantage of using qubits or ones, zeros and superposition of ones and zeros. Certain difficult predictive analytics tasks that have long been thought impossible or intractable for classical computers can now be achieved quickly and efficiently with quantum computing.
Quantum computing will be faster in an environment where there is the need for Markov Chain Monte Carlo (MCMC) based Bayesian framework with large datasets to generate large hierarchical models that require integrations of hundreds or even thousands of unknown parameters. So also will quantum computing be friendlier with complex machine learning algorithms. The Grover’s search algorithm, Shor’s factoring algorithm and the rapid advances in D-Wave’s processors are affirming the possibilities of high level reasoning in decision making processes that are in the pipeline for the next big future in predictive analytics with statistical learning algorithms and machine learning algorithms.
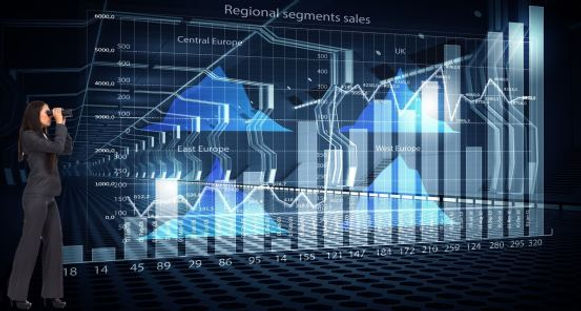
What Can Predictive Analytics Do for A Company that Wants to Break Out and Stay Ahead of the Limitations of the Industry?
Predictive Analytics possesses the know-how on how isolate the different factors (products, markets, customers, volatility, processes etc.) whether as dependent or independent factors on why, when, how these factors are to be included in the strategic plan and implementation plan for the business leaders to create, renew, evolve, coordinate, deploy and recombine resources as the basis of ensuring superior and sustaining competitive advantage (Henderson and Cockburn, 1994; Teece et al., 1997) in a constantly changing and challenging business landscape.
Based on the above, in assessing the opportunities for a company to break out and stay ahead of its competitors, predictive analytics techniques can be applied to:
-
Enhance the dynamic capabilities of a company
-
Advance the adaptiveness for a company to break out
-
Augment the absorptive capacity
-
Create opportunities for innovative breakthrough
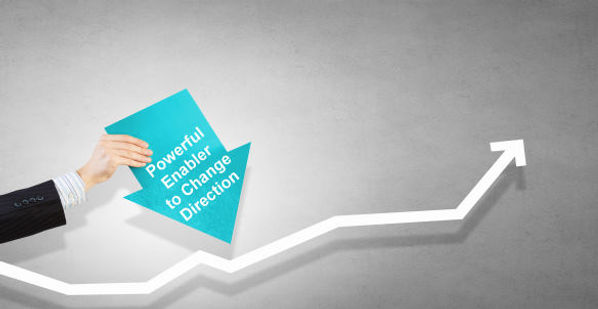
Predictive Analytics Can Enhance the Dynamic Capabilities of a Company
A company that wants to break out and stay ahead of the competition must use strategic plans to support a dynamic fit of what the market wants with what the company has to offer. To make this happen, the leadership must position the company “to create, renew, evolve, coordinate, deploy and recombine resources” as the basis of ensuring superior and sustaining competitive advantage as part of the dynamic capabilities (Henderson and Cockburn, 1994; Teece et al., 1997; Foss et al., 2009) in a constantly changing and challenging business landscape.
The capabilities must allow the company to develop new products and new processes that allow the company to effectively respond to the needs in the market (Helfat, 1997). So also, in order for the capabilities to lead to competitive edge, the company has to combine the resources in configurations (Eisenhardt and Martin, 2000) that are difficult to imitate (Griffith and Harvey, 2001) to be able to effectively manage variability and uncertainty in the market so as to meet the needs of the customers.
Many at times, the capabilities that are expected to make a company very successful can easily become so rigid that they are just another obstacles for the company in attempts to maneuver in a competitive environment. So, dynamic capabilities has to be flexible to allow the companies to make decisions that are unique to them or as responses to the uniqueness of their industries. Volberda (1996) contended that just having dynamic capabilities is not enough but given the hypercompetitive environment, a serious company needs to be quick, bold and experiment in ways that do not conform to the traditional ways of how things are done in the industry. Being flexible allows a company to be able to rapidly respond to different types of changes in timely manner irrespective of the drivers or sources of change. The flexible dynamic capabilities within the context of this write-up include those actions that the leadership has to engage in immediately and the actions that have to be strategically planned to sustain the company once the company breaks out. These are:
-
Immediate Action Plan to Be Implemented
-
Strategic Plan to Enhance the Dynamic Capabilities and Sustain Them
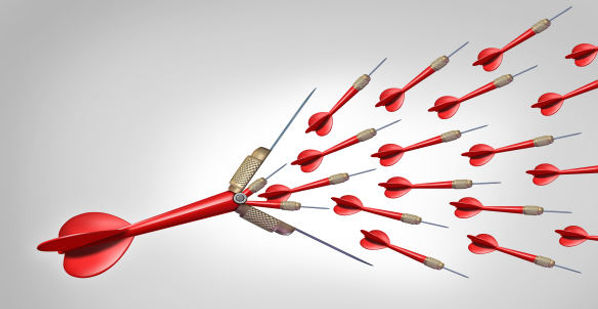
Part I: Immediate Action Plan to Be Implemented
Some of the Immediate Actions – Tactical: 0-6 Months Action Plan
Step I:
-
Assessment of the current performance of the core products and services within the context of delivering to the customers
-
Assessment of the current performance of the core products and services in the market
-
Assessment of the current processes, syems and people to support performance goals of the products and services
-
Assessment of the current market in terms of:
-
Value chain analysis
-
Ranking segmentation in terms of revenue and profit as it may apply to segmentation groups that include but not limited to:
-
Profitability segmentation
-
Market segmentation
-
Geographic
-
Demographic
-
Benefits
-
Psychographic
-
-
Customer segmentation
-
Behavior
-
Loyalty
-
Buyer purchasing power
-
Lifecycle
-
-
-
Critical assessment of the fundamental assumptions of go-to-market strategies
-
Critical assessment of Social Media model and Digital marketing strategies
-
Evaluation of the competitive landscape in terms of competitors’ strength and opportunities to leverage technology, cost advantage and other competitive advantages
Step II:
Based on the above:
-
Realign the current core products and services based on where they are more advantaged in terms of:
-
Changing realities with what the customers want
-
Market realities that cover the features, technology, quality, reliability and cost to deliver
-
Anticipating the future realities in technology development, competitors’ reactions, what to put together to win
-
-
Reposition products and services in light of understanding the customers and the competitive landscape
-
Overhaul go-to-market approach if the approach is inadequate
-
Match resources to
-
Realities with the customers
-
Geographic opportunities
-
Market realities
-
Anticipate the future realities
-
-
Hire the right talent
-
Leverage internal collaborations within the industry and external collaborations outside the industry
-
Reconfigure cost base for example
-
Identify health of assets in order to:
-
Have better insights about the functioning of the assets so as to avoid outage
-
Minimize possibility of down time
-
Reduce part inspection frequency
-
-
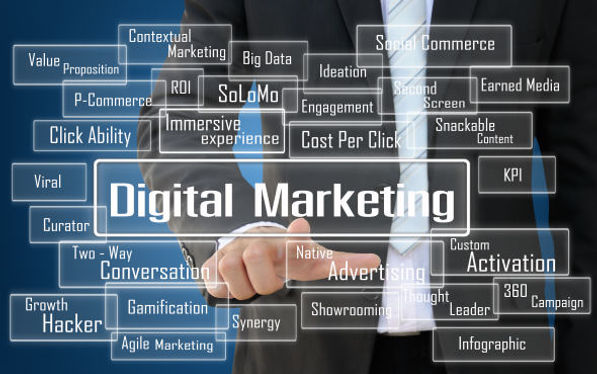
-
Revamp the entire digital strategies in areas of:
-
Digital technologies that include customer database, technologies supporting social media and search engine marketing
-
Digital marketing strategies that include digital delivery and customer relationship management in attraction, acquisition, retention and engagement
-
Building of digital assets
-
Digital engagement and digital marketing delivery mix:
-
Customer Relationship Management
-
Customer engagement
-
Brand awareness
-
Channel optimization
-
-
Search Engine Marketing
-
E-mail marketing
-
Social Media marketing
-
Content marketing
-
Mobile arketing
-
Web traffic strategy
-
Pay Per Click (PPC) services
-
Digital database and analytics
-
-
From Operations Analytics through Marketing Analytics to Predictive Analytics
The analytics to support immediate actions can come from Operations Analytics which will provide analytical assessment of the current performance of the core products and services, the analytical assessment of the current processes to support the products and services performance goals. Marketing analytics, part of enterprise analytics, can support the assessment of the current market in terms of value chain analysis, market segmentation, customer segmentation, the fundamental assumptions of the go-to-market strategies, the competitive landscape in terms of competitors’ strength and opportunities to leverage technology, cost advantage and other competitive advantages.
The predictive analytics can provide insights not only on how the products and services will perform, but also can create enhancement that will result in optimal performance through providing the scoring of customers, and for example, the scoring of which phone numbers to call in the loan collection call center, the scoring of the customers to call to collect the loan, the scoring of the employee to take the phone, scoring of the employees’ performance and account scoring. The creation of scoring models allows the business to focus the work efforts. For example, work efforts are directed on customers, accounts, phone numbers that score higher in the loan collection call center.
Predictive modeling will also help in providing what should be the goal for the day whether in call center, in sales, in production, in productivity and revenue.
Some of the areas that can be supported by predictive analytics in implementing Immediate Action Plan include:
-
Develop scoring models and leverage scoring algorithms where appropriate for managing the current core business products and services to include:
-
Portfolio performance score model
-
Account performance score model
-
Customer scoring model
-
-
Develop models using algorithms to capture factors in market realities for the current core businesses
-
Predict the trajectories of the current products and services and compute scenario modeling for the repositioning of products and services
-
Perform predictive competitive profiling based on customer and market information from big data source(s)
-
Develop explanatory and association models to match new products and services as off-shoot of current products and services in terms of:
-
Realities of the customers
-
Geographic opportunities
-
Market realities
-
Anticipate the future realties
-
-
Develop predictive decisioning models including using algorithms to identify the probable factors for the future business in terms of:
-
Sales of new products
-
Effectiveness of marketing campaigns
-
-
Change go-to-market approach – Leverage Operations Analytics to reduce late-cycle product and service design, changes, product delays and improve design decisions and develop model to capture seasonality as an effect in go-to-market.
Predictive modeling and predictive profiling algorithms can be used to profile the customer or transaction profiling in areas that include:
-
Customer Selection Model
-
Recency, Frequency, and Monetary Value (RFM) model
-
-
Customer Acquisition Model
-
Customer Development
-
Customer Retention
-
customer Engagement
-
Customer Life Time Value
-
Customer Profitability Model
-
Churn Prediction Models
-
Market Basket Association Model
These Immediate Action Plan recommendations coming from enterprise analytics like operations analytics and predictive analytics can be enhanced by generating scenario analysis which focus on “what-if analysis’. In making decisions, the predictive modeling can outright support the decisions the business leaders want to make if the level of uncertainty is low or very manageable. Scenario analysis can be used to ask the question of “what if”. In case there is medium to high uncertainty, simulation modeling can be included as this allows the leaders to have some elements of control. The controlled factors can be called decision factors. In the face of uncertainty and complexity, the need to have these decision factors may still not be enough. The uncertainty and complexity imply that there are hundreds or thousands potential alternative decisions.
Optimization can help to find the optimal values. Optimization has certain elements to it. This includes the decision factors (You have control), the constraints (what limits the values of the decision factors), variables, assumptions (uncertainty of the model) and the objective (Desired outcome or forecast). Optimization includes searching for the optimal values.
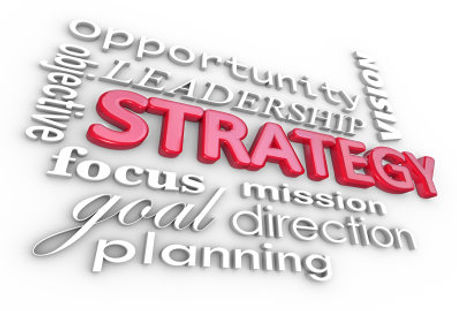
Part II: Strategic Plan to Enhance the Dynamic Capabilities
Some of the Long Term Actions – More than 6 Months Actions Plan
Below are some of the strategic questions that can elicit insights to develop the dynamic capabilities. These include:
Where is the Company Now?
-
Where is the company, among others, in terms of scale and presence, brand strength, market performance, quality and composition of earnings?
-
Where is the company in term of maturity curve?
-
Where are the products and services in terms of their life cycles?
-
What are the different maturity curves for the different product lines and service lines? This question has importance not only for the deliverables of relevant predictive models and predictive profiling but also scoring models that can be put together to score accounts, customers in terms of churn, retention etc. The scoring of account performance in the early stage will probably not work very well if applied to old accounts.
Where are the Competitors Doing Now?
-
In comparison with the industry, where is the industry in terms of maturity curve?
-
What are the dominant factors governing the tendencies within the industry?
-
How will the competitors redefine their activities?
-
How will the competitors’ functional strategies change?
-
How will the competitors change their investment strategies?
Where does the Company Wants to Go?
-
Where does the company wants to be, among others, in terms of scale and presence, degree of customer sophistication, knowledge of customer expectations, size in segments served, product positioning, sales level, revenue and profitability?
-
How will the financial performance be affected?
-
How will the change in strategic direction impact among others the widespread/geographic spread, rapidity of penetrating new markets, increase in cash flow, closeness to the customers, long-term continuity with the customers and broadest possible perspective of a targeted market?
How will the Company Get There?
How will the company get there, among others, in terms of what are the options for the companies regarding marketing decisions, product decisions, product-market strategy decisions, pricing decisions, promotion decisions, distribution decisions, communication decisions and customer service decisions?
Alignment of the Predictive Analytics Deliverables in the Strategic Management Process
Leveraging Predictive Analytics:
Predictive Analytics adds tremendous value at three levels of strategic planning which include:
-
Corporate Level
-
Competitive Level
-
Functional Support Areas Level
Strategically align the eight different predictive analytics techniques to generate enormous returns for the business for example:
-
Leverage predictive analytics to identify and sustain new business/service model with increasing revenue streams from innovative driven products, services and processes that benefit the customers
-
Deploy predictive modeling as enabler to increase the speed to market with new products and services that the customers want, where they want the products and services, how they want the products and services and ready to pay for the products and services in such a way that the company is profitable and ready to invest in designing and developing new generations of products and services with high value content for the customers
-
Integrate predictive profiling as the best internally consistent methodologies to profile the customers to find out what they want and keep them coming back for more, profile the systems and processes to support what the customers want and profile the competitors so as to deliver better value than them to the customers
-
Enhance the decision making process even within the context of uncertainty and still make the optimal decisions with predictive decisioning
-
Leverage MPC to optimize the production processes and operations, driving automation of processes and delivering higher product quality
-
Leverage real-time sensor-driven predictive maintenance to prevent costly downtime
-
Learn constantly from the experience, improving based on the learning and making the best choice with predictive reinforcement and predictive representations
-
Leverage algorithms to track behavior and monitor activities to offer customers arrays of products, suggestions on their searches, allow them to compare products, pull up reviews of people who have bought the products before so that they can buy and buy more than they thought they want to buy with predictive search and recommender systems
-
Improve operational efficiency and making it easier for the employees to effectiveness and efficiency access valuable indexed information to drive strategic initiatives that provide competitive edge in winning and retaining profitable customers with predictive coding
At the strategic planning levels, all the eight techniques will have to be used. Predict Analytics is an embodiment of what the business leaders need to best leverage strategic opportunities in maneuvering through the changing competitive environment. Predictive Analytics offers the leadership to continually reevaluate the strategy based on perceived opportunities, existing and emerging threats from the external environment. Predictive analytics can identify the organizational strength factors that can help and internal weakening factors that can hinder in attempts to develop and leverage competitive advantages. These areas include:
1. Corporate Level
This involves working with the Strategic Management Office, Strategic Operations and leadership teams in anticipation of business opportunities, including growth, expansion, market realignment, mergers and acquisitions etc. and how best to predict what factors the company can control to effectively respond and ensure continuation in being in control within the context of re-evaluating and realigning strategic options to outpace competitors which include:
-
Enhancement of Value Proposition
-
Transforming from building products and services value to building customer value through customer focus, customer satisfaction, customer loyalty and customer service. Essentially transforming from product centric to customer centric value proposition.
-
Service innovation in product development and offerings
-
-
Enabling Strategic Innovation
-
Acquisition, adaptation and implementation of new innovative technologies
-
Innovation drivers
-
Open innovation
-
-
Crowdsourced product and marketing ideas
-
Crowd collaboration
-
Acquisition and implementation of improved production methods
-
Alignment in process design, re-design and improvement
-
Emerging analytics, digital analytics, algorithms, social and data science technologies that include statistical learning algorithms, machine learning algorithms and optimization.
-
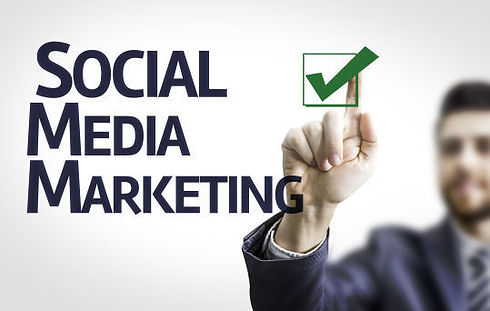
-
Driving Strategic Digital Customer Model
-
Digital Enterprise Model
-
Digital platforms
-
Scalable platform
-
-
-
Digital technology infrastructure
-
Digital supply chain
-
Digital logistics
-
Digital engagement
-
Digital user, owner and customer experience
-
Communication channels interactions
-
Email campaign strategy
-
-
Digital payments
-
Brand awareness
-
Affiliate networks
-
-
Search Engine Optimization
-
Pay Per Click (PPC) advertising
-
Viral marketing
-
Web conversion optimization
-
Social media model
-
Social network strategy
-
Content creating and sharing
-
-
Mobile
-
Adaptiveness to changing technical advancement capabilities
-
Location services
-
Messaging services and marketing
-
Mobile services with authentication
-
Smart mobile apps
-
Mobile web
-
-
Cloud
-
Cloud based applications and solutions
-
APIs integrations
-
Sourcing opportunities
-
-
-
Transforming Strategic Business Performance
-
Leveraging the vision of what customers want and how best the company can best deliver value to the current and future customers
-
Simplification of offerings configurations
-
Satisfying consumer demand
-
Targeting and optimizing adverts
-
-
Business units’ performance
-
Integration of the service model
-
Shared deliverables, workspaces, virtual teams, and virtual organizations
-
-
-
Aligning the Strategic Financial Performance with the Customer Centricity
-
Predicting earnings performance
-
-
Strategic Marketing Performance in terms of:
-
Establishment of new business
-
Target market segments
-
Existing product and services performance
-
New product and services performance
-
Products and services – The markets where these products and services can have the highest returns
-
Who are the customers buying in these markets and what to do to keep the customers coming back in terms:
-
Customer acquisition, churn, retention, customer lifetime value (CLV)
-
Measure of referral including Net Promoter Score (NPS) and satisfaction index
-
Profitability score of the customers
-
Customer complaints
-
Customer turnover rate
-
Customer buying behavior in terms of psychology, culture, subculture, family decision making, perception, attitudes etc.
-
-
-
Market segmentation
-
Product and services targeting and positioning
-
Cross-selling opportunities
-
Information search and decision Making
-
-
Returns on advertising campaigns
-
Service/product positioning
-
Cost and/or value leverage
-
Strategic digital Marketing
-
-
Strategic Operations Performance
-
Embedded technologies
-
Predictive maintenance
-
-
Strategic capacity performance
-
Capacity utilization
-
Inbound and outbound logistics
-
Inventory management
-
Supply chain management
-
IT performance
-
Procurement
-
Human resources management that include hiring, retaining, training, employee productivity, employee satisfaction and employee loyalty
-
Virtual and digital workspace
-
-
-
Enterprise Risk Management
-
Strategic risk management, Asset-Liabilities risk management, Credit and/counterparty risk, Liquidity risk, Operational risk, Portfolio risk, Compliance risk, Marketing risks, Pricing risk, Balance sheet composition risk, Underwriting risk and Reserving risk
-
-
Capital Management
-
Adequacy of capital
-
Risk to capital
-
Capital at risk sensitivities
-
-
Emerging Risk Threat
-
Ability to respond to volatility in the market
-
Agility in being able to manage unexpected rapid change
-
Integrating the company strength while maximizing the opportunities to mitigate active and inactive threats
-
2. Competitive Level
-
How best can the company create value as the base to compete through:
-
Developing new processes of delivery
-
Improving on existing products or/ and develop new products that the customers value
-
Adding new services to existing products
-
Adding services to the new products
-
Developing or offering standalone products and services
-
-
Assess and leverage industry value drivers for example in terms of the cost and differentiation in product and service offerings
-
Identify the specific activities and processes that can enable the company to create unique value that represents competitive edge for the company in its market with current and new customers
-
Identify the factors that can best align the company’s activities so all leadership efforts or at least most of the efforts can consistently reinforce the current and potential advantages in support of current competitive positioning and future repositioning.
-
Lowering the cost structure without impact on product and service quality
-
Anticipate and leverage disruptive technologies
-
Competitors’ moves in term of alternative competitive strategies
3. Functional Support Areas Level
-
Work with the Functional Support Areas to reinforce the company’s internal resources that can ensure the successful implementation of competitive strategy.
-
Work with the different areas to provide predictive outlooks to support the objectives for marketing, finance, research, technology, operations, and so on to include:
-
Cost performance
-
Technology performance
-
Employee performance
-
Internal operations performance
-
-
Identify factors through predictive analytics of activities and processes that can help the company to be able to maximize its competitive positioning. These include:
-
Support process performance
-
Support service performance
-
-
Identify factors through predictive analytics of how best the functional Support areas fit with the company’s strategy to effectively compete.
-
Work with the Support areas to use predictive analytics to anticipate and leverage disruptive technologies
-
Work with the Support areas to provide predictive analytics resources to support the response to the competitors’ moves in term of alternative competitive strategies.
-

Predictive Analytics Advances the Adaptiveness for a Company to Break Out
Companies with adaptiveness have the capacity to adjust to different types of changes emanating from the market, ancillary industries, competitors, clients, partners and customers in order to be able to maintain its viability (Miles and Snow, 1978).
In addition, the company is able to anticipate problems, and be able to keep up with changes and new ways not only with the changes in products and services but also with changes in business model, revenue model, marketing model and profitable customer generation model.
Adaptiveness of the company also includes the ability to analyze a wide variety of opportunities not only improvement but also becoming a company that can strategically break out of the mode, eliminate or overcome constraints, modify, reconfigure resources, develop new capabilities, enhance competences, quickly read and interpret the market, adapt and eventually lead the industry.
Leveraging Predictive Analytics: An adaptive company that is focusing on performing better than any of its competitors will find that predictive analytics is an enabler. Predictive analytics can provide in-depth support that an adaptive company needs to be able to respond to unknown factors that can impact the trajectory of the strategic plan especially during implementation based on new emerging business opportunities. With predictive modeling, new and emerging business opportunities can be identified, discerned, measured with proper metrics that are provided to business operations leadership and handed over to the Operations Analytics Team, Risk Analytics Team or Decision Support Analytics Team to monitor and provide Action Plan while the BI Reporting Analytics Team provides the Intelligence reports with progress on changing factors as part of the Action Plan Updates that support operation performance. Deliverables for predictive analytics team is the ability to identify active and passive factors that can enhance the robustness of the company’s strategic plans and implementation to quickly adjust, respond and reallocate its resources to take advantage of the changing market direction, customers’ need, pricing movement, new technologies and leverage them as “emerging market opportunities” (Chakrovarthy, 1982; Foss et al.).

Predictive Analytics Augments the Absorptive Capacity to Commercialize Knowledge
A company that wants to break out of its industry and take the lead must have the ability to recognize the value of new external information, assimilate it, and apply it to commercial ends knowledge (Cohen and Levinthal, 1990:128). The company must be able to integrate external knowledge with the internal knowledge in such ways that it does not generate unmanageable conflicts and does not begin to create a new silo or reinforce the existing silos. The assimilation of the new knowledge should be an opportunity for the company to break down the silos if they exist, otherwise, it will be very difficult to bring together the different departments to work on the new information in a go-to-market process.
Operationalizing absorptive capacity within a company is marked by four stages: acquisition, assimilation, transformation, and exploitation of market knowledge (Zahra and George, 2002).
In the first stage stage, the acquisition stage, the company must be able to identify what type of information it is seeking for. Is the information about the trend that involves the:
-
Competitors?
-
Customers?
-
Clients?
-
Market?
Where are the information likely going to be located and how does the company go around collecting them?
Collection and indexing are important elements that the allow other people within the company to have access to the information.
The second stage, the assimilation stage, includes the recognition and understanding of the collected information. Based on the information, the company can begin to ask the questions that directly involves gap analysis. This provides opportunity for the business to ask the question whether many of their current products, services and processes can be compared with the industry.
The third stage, transformation stage, is where the company begins to take the output from the gap analysis to be able to convert them to opportunities.
The fourth stage, the exploitation stage, allows the company to turn the information into a commercial endeavor.
Predictive Coding: The algorithms in predictive coding helps to classify and sort document including emails, scanned and electronic legal documents and behave like a legal reviewer. Algorithms driven indexing of information on the competitors, customers, clients and market are important information that are needed to be made available to other people within the company to assimilate and apply to commercial ends. Indexing the information with predictive algorithms makes it easier for accessibility.
Predictive analytics augments the ability of the company to be able to absorb external knowledge (Cohen and Levinthal, 1990) from ancillary industries, competitors, clients, partners and other learning sources and integrate with internal knowledge so as to deliver new superior level of performance from the business.
For instance, in an attempt to predict attrition trend and given the consequences it will have for successful implementation of the strategic plan, predictive analytics is able to go beyond depending on internal factors alone. Rather, predictive analytics leverages the sentiment analysis (unstructured data) format that is being used in predicting customers’ churn as a format to capture the ex-employees’ and industry employees’ sentiments expressed on indeed.com, glassdoor.com and other recruiting sites.
The sentiment data is combined with the internal factors including the internal sentiment data collected from the Employee Opinion Surveys, Employee Engagement Surveys and employee net promoter score (eNPS) and freely shared email from individual employee and/or group of employees.
The output is not only more robust in terms of the predictive output showing what the attrition trend will look like, its impact on the strategic plan, what can be done to reduce attrition rate, who and where are the people the company considers critical to the success of the plan, who should be encouraged to stay, who should be encouraged to leave and how best can the business manage attrition in its quest to implement its strategic plan successfully.
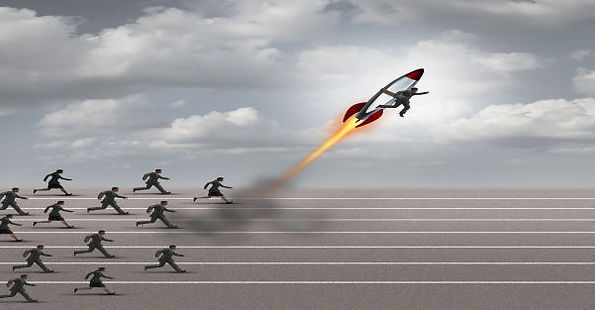
Predictive Analytics Creates Opportunities Innovative Breakthrough
In most of the industries, technological changes are evolving at warp speed. Predictive analytics is capable of understanding the rapid evolution but it can also integrate new innovative ideas into analytics deliverables that can enable major changes in strategic plan for the company to have competitive edge and be able to break out of its industry’s limitations.
Business leaders can integrate emerging predictive analytics algorithms, breakthrough models and scoring models as sources of revenue, basis for driving market innovativeness and ensuring technological sophistication in the delivery of their products and services (Capon et al. 1992). Many smart companies with the right skillsets are being positioned to leverage predictive analytics to effectively and efficiently improve their current inventory of products, processes and services and also leverage the predictive analytics in its innovation deployment strategies. Some of the pre-requisites for innovative breakthrough that predictive analytics can add value include:
-
Identifying opportunities
-
Understanding the customers
-
Designing and building new products and services
-
Leveraging opportunities from value chain
-
When to seize opportunities to commercialize based on the changing landscape in the industry.
In areas that include:
-
Strategic Digital Customer Model
-
Digital Enterprise Model
-
Digital platforms
-
Scalable platform
-
-
-
Digital technology infrastructure
-
Digital supply chain
-
Digital logistics
-
Digital engagement
-
Digital user, owner and customer experience
-
Communication channels interactions
-
Email campaign strategy
-
-
Digital payments
-
Brand awareness
-
Affiliate networks
-
-
Search Engine Optimization
-
Pay Per Click (PPC) advertising
-
Viral marketing
-
Web conversion optimization
-
Social media model
-
Social network strategy
-
Content creating and sharing
-
-
Mobile
-
Location services
-
Messaging services and marketing
-
Mobile services with authentication
-
Smart mobile apps
-
Mobile web
-
-
Cloud
-
Cloud based applications and solutions
-
APIs integrations
-
Sourcing opportunities
-
-
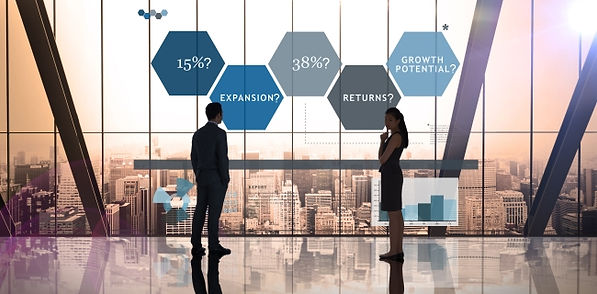
Best Practices Roadmap to Leverage Predictive Analytics Within the Corporate Environment in terms of:
Strategic Responsibilities of the Executive and Senior Leadership Teams
-
Create and deploy the Enterprise Analytics/Analytics/Predictive Analytics/Data Science Department and provide executive support and sponsorship for predictive analytics as part of the vision of the company so as to overcome organizational barriers
-
Drive the changes needed for data- and analytics- driven culture
-
For the best value realization, integrate predictive analytics leadership and team as part of the Strategic Planning Process and treat predictive analytics as a business capability
-
Leadership has to emphasize that rewarding Strategic Planning may be boring and that predictive analytics is not financial projection
-
Organizationally align analytics including predictive analytics to be part of Strategic Operations Team
-
Avoid unnecessary shortcut: buying off-the-shelf predictive and scoring models instead of developing proprietary predictive and scoring models
-
Run Predictive Analytics Team as an internal consulting team and as a prerequisite to become a Strategic Business Unit (SBU)
-
Avoid an uncompetitive loop in the Industry Box
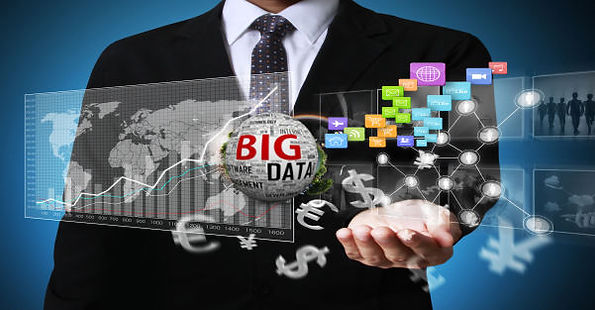
1. Create and Deploy the Enterprise Analytics/Analytics/Predictive Analytics/Data Science Department and Provide Executive support and sponsorship for predictive analytics as part of the vision of the company so as to overcome organizational barriers
The Board, the Executive Team and the Senior Management Team will have to provide support for the creation and deployment of the Enterprise Analytics/Analytics/Predictive Analytics/Data Science Department and provide executive support and sponsorship as part of the vision of the company so as to overcome organizational barriers.
The executive leadership has to be involved enough to be able to provide rationale and justification of how the predictive analytics deliverables will enhance the company performance and be beneficial to the employees and the customers.
Predictive Analytics as a team, process or even an internal consulting team cannot exist on its own, it has to be intelligently handled as enabler to support the company’s strategy. Predictive Analytics is not going to perform very well where the business leaders cannot or unwilling to put together a serious and sustainable business strategy. No matter how advanced the automobile GPS navigation is, if there is no destination selected, the automobile GPS navigation is just part of an interior decoration of a car.
Just like the automobile GPS, once a direction is entered, or once predictive analytics is leveraged as part of strategic planning, deployment, implementation and measurement in the business, then the business leaders will see their company as empowered forward looking and ready to out compete business operations
2. Data- and Analytics- Driven Culture
Pursue a data- and analytics- driven culture within the senior and executive leadership teams. Use data and analytics as the basis for driving daily, weekly, monthly and quarterly business reviews that were predicted at the beginning of the Annual Strategic Business Reviews. This culture understands and leverages analytics best practices to measure the impact on business results based on the use of data streaming and event processing. It also seeks and uses different types of predictive analytics best practices to align with business strategies as the basis to set the measurable limits on impact of business results in the areas that include:
-
Monthly Reviews and Action Plan
-
Quarterly Reviews and Action Plan
-
Annual Strategic Planning
-
Account Review (Transaction)
-
Growth Playbook
-
Annual Portfolio Review (Annual Portfolio Risk Reports)
-
Capital Management (Economic Capital, Capital Allocation and Capital Requirements)
-
Different types of benchmarking analytics that included:
-
Comparative Benchmarking (Comparing the company for internal Improvement)
-
Strategic benchmarking, Process benchmarking, Operational benchmarking and Risk Management benchmarking
-
-
Competitive Benchmarking (Benchmarking to seek Competitive edge or advantages)
-
Risk to Strategic Plan benchmarking, Risk to Rating benchmarking, Product Performance benchmarking and Market Performance benchmarking
-
-
This cultural approach becomes the basis for change that enhance the leadership’s ability to drive the change down the organization
3. For the Best Value Realization, Integrate Predictive Analytics Leadership and Team as Part of the Strategic Planning Process and Treat Predictive Analytics as a Business Capability
A company looking to break out and stay ahead of its competitors has to integrate Predictive Analytics Leadership and Team as part of the strategic planning process. In many companies, this team is either not represented enough or not even represented at all.
Given the fact that strategic plans are designed as forward looking to be executed within a year, 3 years and 5 years, it is rather strange that predictive analytics team which has the capability to see into the future is not an active member of the strategic planning process.
4. Leadership Has to Emphasize that Rewarding Strategic Planning May Be Boring and that Predictive Analytics is not Financial Projection
Putting together good strategic plan may involve going through the “boring” steps of pre-planning, planning, deployment, implementation, measurement, monitoring and reporting. As “boring” as these steps are, they are very rewarding in terms of increasing market share, productivity, revenue and profit.
Many business leaders like to see financial projection as the “plan”. No matter how well a financial projection is designed, it is still inadequate.
Financial projection tends to focus on narrow conception of threats and responses to revenue and expenses derived from historical events. Outcomes tend to be described in terms of consequences which can easily be measured and monitored in terms of the biggest share of expenses – Headcount.
There are many cases that the managing of financial projection to plan is also a short hand to managing the biggest share of expense in operations, the headcount.
The numbers in the financial projections may look good during the financial projection sessions but the reality is that by the beginning of fourth quarter, the variance tends to come in higher than planned and because the biggest share of expenses is headcount, the default for business leaders is to figure out how to manage or reduce expenses which in other word, reduce headcount.
A company that wants to break out of its industry’s limitations must spend the time in putting together the strategic plans. For the business leaders who are familiar with art of warfare, the financial projection is more like having one of the branches of the military and going to a full blown war with just one branches of the military is outright reckless. Having a strategic plan include all the military power to win the war but also win the peace!
5. Organizationally Align Analytics including Predictive Analytics to Be Part of Strategic Operations Team
Unless the company is in IT related industry, Predictive Analytics or Analytics Team do not belong in IT or Finance Departments! It is part of Strategic Operations! And if Predictive Analytics or Analytics Team is in IT related industry, it has to be part of IT Strategic Operations!
Before Big Data Analytics, IT Department was the data custodian. Today, with the need for faster access to data to make decision, this is one of the trickiest issue that can cause friction between IT leadership and Analytics leadership. There are many alternative ways to resolve this issue but the solution is not integrating Analytics Department as part of IT Department because most of the Analytics deliverables are to provide result-oriented support for the Strategic Business Operations to execute and deliver.
For IT related companies or industries, Analytics Department has to be placed in the Strategic IT Organization where analytics can directly impact growth in market share, revenue and profitability. For industries like Financial Services, Healthcare, Logistics, Retails (online and Brick and Mortar), Predictive Analytics Team and Analytics in general has to be part of Strategic Operations reporting to the CEO or the COO at a minimum.
6. Avoid Unnecessary Shortcut: Buying off-the-shelf Predictive and Scoring Models instead of Developing Proprietary Predictive and Scoring Models
Business leaders have to make decisions on “buy versus build” on many occasions.
One of these decisions that the business leaders should be able to make easily is to build proprietary predictive and scoring models. There is no doubt that many off-the-shelf Predictive and Scoring Models are very good. For many of the business leaders that do not want to wait to build, they can plug and use off-the-shelf predictive and scoring models which to them may look attractive as the opportunities to enhance the performance of the businesses.
If a company has serious intention to break out of the limitations of the industry, then this is not a very smart way to go. Non-proprietary predictive models and scoring models are based on the industry data which in fact translates to using predictive models and scoring models based on data that is the industry’s average. Accepting to adopt industry’s average built predictive models and scoring models is also accepting that the company has no competitive advantage and that the business leadership is content to perform at below average or at best at average in the industry.
Generally, there are little upward bumps that follow the plug and use of these non-proprietary predictive models and scoring models which is a reflection that the company was really performing below the industry's average. As the competitors raise their performance, so also the resumption of the downward trend. The company with non-proprietary predictive models and scoring models is following the industry and there is no way to outperform the industry.
For a sustainable competitive edge, business leaders have to accept to build proprietary models and scores that can outperform the industry.
As already pointed out, the non-proprietary predictive models and scoring models or off-the-shelf predictive and scoring models are very good but these should be seen as input into building of the proprietary predictive models and scoring models. There are unique business factors that have to be integrated into proprietary predictive models and scoring models which can be combined with the industry factors so as to give the edge to the company to outperform the industry.
If a company buys industry’s average sentiment scoring model as a way of reducing or managing its attrition rate, the non-integration of the sentiments in the company’s employee surveys by the current employees and the sentiments of the ex-employees left in places like indeed.com and glassdoor.com will make the scoring model inadequate for the business leadership to manage the uniqueness of it attrition rate issues.
7. Run Predictive Analytics Team is an Internal Consulting Team as a Prerequisite to Become a Strategic Business Unit (SBU)
There are numerous surveys that show that is difficult to retain the members of Predictive Analytics Team. Many members of the team are out to solve strategic problems but most of the time, the business problems are tactical. So many of them are confronted with deliverables that are of “Fix or Repair Daily” deliverables.
One of the obvious solutions to retain them include giving them the innovation, collaboration, design experimentation and algorithms development opportunities to build data platforms, analytics solutions, predictive analytics as a service, applications, patents, intellectual property, algorithms driven solutions that can be commercialized and let them have a share of the benefits of the commercialization.
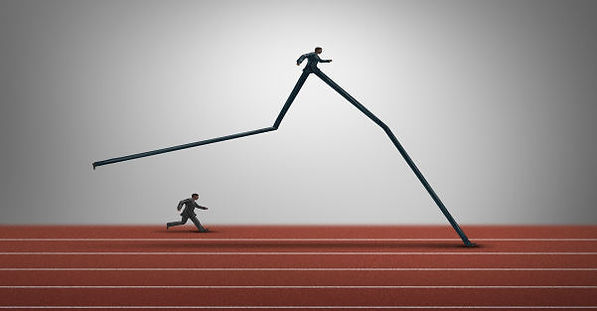
8. Avoid an Uncompetitive Loop in the Industry Box
Predictive Analytics deliverables should not be designed to help the company fight the competitors as if in a boxing match. Predictive Analytics is to increase the company’s speed, insights, agility and innovative power to unlock new opportunities to leave the competitors behind.
Predictive Analytics helps to:
-
Identify and sustain new business/service model with increasing revenue streams from innovative driven products, services and processes that benefit the customers -Predictive Analytics
-
Increase the speed to market with new products and services that the customers want, where they want the products and services, how they want the products and services and ready to pay for the products and services in such a way that the company is profitable and ready to invest in designing and developing new generations of products and services with high value content for the customers - Predictive Modeling
-
Profile the customers to find out what they want and keep them coming back for more, profile the systems and processes to support what the customers want and profile the competitors so as to deliver better value than them to the customers – Predictive Profiling
-
Enhance the decision making process even within the context of uncertainty and still make the optimal decisions – Predictive Decisioning
-
Optimize the production processes and operations, driving automation of processes and delivering higher product quality – Model Predictive Control
-
Leverage real-time sensor-driven predictive analytics to prevent costly downtime – Predictive Maintenance
-
Learn constantly from the experience, improving based on the learning and making the best choice – Predictive Reinforcement and Predictive Representations
-
Leverage algorithms to track behavior and monitor activities to offer customers arrays of products, suggestions on their searches, allow them to compare products, pull up reviews of people who have bought the products before so that they can buy and buy more than they thought they want to buy– Predictive Search
-
Improve operational efficiency and making it easier for the employees to effectiveness and efficiency access valuable indexed information to drive strategic initiatives that provide competitive edge in wining and retaining profitable customers - Predictive Coding
Best Practices Roadmap to Leverage Predictive Analytics Within the Corporate Environment in terms of:
Strategic Responsibilities of the Chief Analytics Officer/SVP and Predictive Leadership and Deliverables
Internal facing deliverables for the Analytics/ Predictive Analytics Team and Organization
-
Predictive Analytics Strategic Overview
-
Predictive Analytics Governance Model
-
Predictive Analytics Operating Model
-
Predictive Analytics Organizational Model
-
Predictive Analytics Deliverables Overview
External customer‐facing deliverables to support the business strategic performance and goals
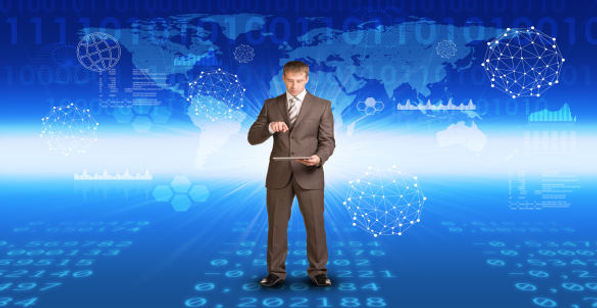
Internal Facing (the Internal Predictive Analytics Team)
1. Predictive Analytics Strategic Overview
Leverage the Enterprise Analytics Strategic Framework or establish predictive analytics strategic framework to include:
Strategic Planning
-
Set clear mission and objectives
-
Develop and implement strategic requirements to develop the relevant capabilities
-
Develop strategic operating plans that reflect the longer term objectives and priorities in maximizing the capabilities
-
Strategically align the eight different predictive analytics techniques to generate enormous returns for the business for example:
-
Leverage predictive analytics to identify and sustain new business/service model with increasing revenue streams from innovative driven products, services and processes that benefit the customers
-
Deploy predictive modeling as enabler to increase the speed to market with new products and services that the customers want, where they want the products and services, how they want the products and services and ready to pay for the products and services in such a way that the company is profitable and ready to invest in designing and developing new generations of products and services with high value content for the customers
-
Integrate predictive profiling as the best internally consistent methodologies to profile the customers to find out what they want and keep them coming back for more, profile the systems and processes to support what the customers want and profile the competitors so as to deliver better value than them to the customers
-
Enhance the decision making process even within the context of uncertainty and still make the optimal decisions with predictive decisioning
-
Leverage MPC to optimize the production processes and operations, driving automation of processes and delivering higher product quality
-
Leverage real-time sensor-driven predictive maintenance to prevent costly downtime
-
Learn constantly from the experience, improving based on the learning and making the best choice with predictive reinforcement and predictive representations
-
Leverage algorithms to track behavior and monitor activities to offer customers arrays of products, suggestions on their searches, allow them to compare products, pull up reviews of people who have bought the products before so that they can buy and buy more than they thought they want to buy with predictive search and recommender systems
-
Improve operational efficiency and making it easier for the employees to effectiveness and efficiency access valuable indexed information to drive strategic initiatives that provide competitive edge in wining and retaining profitable customers with predictive coding
-
-
-
Ensure that the operating objectives and standards of performance of the capabilities are not only understood but owned by the Analytics and predict analytics management and employees
-
Drive the strategic orientation of the Predictive Analytics Team and run as a capability for the company
Operational planning
-
Put in place adequate operational planning and control systems so as to closely monitor the operational results from the capabilities against plans
2. Predictive Analytics Governance Model
Leverage the Enterprise Analytics Governance Framework or establish predictive analytics governance framework to include:
-
Predictive Analytics Architecture that includes delivery method, technological requirements, security protocol and control both for the data, modeling and implementation of output
-
Policies and Procedures: Complete the guiding documentation and identify policies and standards for:
-
Current and future activities
-
Standardization of Procedures
-
-
Processes
-
Complete service descriptions
-
Complete point-of-contact process description
-
Lay out user interaction with the internal and external customers
-
Establish the processes to scrutinize the performance in meeting agreed goals and objectives
-
Monitor the reporting of performance. Take remedial action where necessary
-
-
Risk
-
Ensure the integrity of operational information and controls are robust and defensible.
-
-
Quality Tracking
-
Implement reports and scorecards to control and monitor:
-
Design effectiveness
-
Operating effectiveness
-
Quality of deliverables
-
-
-
Performance Measurement
-
Create structures and processes that allow for objective identification and measurement of drivers that can be influenced if there is the need to change the current course of action(s) through Operational Reporting, Dashboards and Scorecards:
-
Provide the Executives with report cards and scorecards on the performance of the organization. Design, develop and increase the use of dashboard across company to:
-
Enhance tactical view for advanced analytics with drill-down capabilities.
-
Display operational data and metrics in near real time.
-
Enhance the company capabilities to monitor enterprise sensors with alert system to ensure quick turnaround on major incidents with issue resolution.
-
-
Monthly Operations Reports (MOR)
-
Provide Monthly review of operations performance
-
-
-
3. Predictive Analytics Operating Model
-
Predictive Analytics Services
-
Define Predictive Analytics solutions and related service offerings, service level expectations, and point-of-contact process
-
Map services to the needs in the business units, support areas and other customers.
-
Identify data sources and data sets
-
Identify core predictive analytics for self-service architecture
-
Define user groups and security access
-
Drive Predictive analytics and performance metrics for profitability: Use advanced predictive analytics methods and algorithms to identify the factors that can drive up business-line profitability
-
-
Core Processes
-
Specify formal processes required to manage the internal and customer facing operations and practices
-
Establish Predictive analytics operating processes that can efficiently be leveraged to provide the needed input for different stakeholders whose responsibilities are different areas of the company that include:
-
Portfolio management
-
Pricing
-
Reserving
-
Sales and Marketing
-
Distribution channels or logistics
-
Customers Relationship Management
-
Client managementt
-
Underwriting
-
Capacity management
-
Volatility management
-
Enterprise risk management
-
Strategic Risk
-
Operational Risk
-
Premium Risk
-
Underwriting Risks
-
Marketing Risks
-
Asset/Liability Matching Risks
-
Underwriting Risks (Pricing)
-
Reserving Risks
-
Credit Risks
-
Market Risks
-
Interest Rate Risks
-
Exchange Risks
-
Liquidity Risks
-
-
Capital Management - (Allocation, Economic, Regulatory and Cost of Capital)
-
-
-
Predictive Analytics Tools Support
-
Complete delivery method
-
Define technological requirements
-
Define and capture analytic metadata
-
-
Quality Tracking - Implement reports and scorecards to control and monitor:
-
Design Effectiveness
-
Operating Effectiveness
-
Quality of deliverables
-
-
Run Predictive Analytics Team is an Internal Consulting Team as a prerequisite to become a Strategic Business Unit (SBU)
4. Predictive Analytics Organizational Model
-
Strategic Staffing Plan: Implement strategic staffing plan that converts the requirements from the business units into strategic requirements for the Predictive Analytics Team in having the right talents, the right level, the job family(ies) and required skillsets. Other areas covered by the strategic staffing plan include development of internal resources, workload management, retention plan, rewards system and anticipated turnover. There are many occasions that the staffing is supplemented by global workforce. The talents that tend to end up in Predictive Analytics Team are global talents, so the competition for the best is on global basis and fierce in terms of recruitment, retention, promotion and development!
-
Roles and Responsibilities Matrix
-
The Team is strategically staffed to leverage the specialized skillsets of these smart individuals rather than convert them to be generic employees for what they were not recruited to deliver.
-
Team Expertize: Assemble the right team to meet the challenge and teaming and coordination will be paramount to achieve results. When planning for a major endeavor, the leadership will need to tap into all the different kinds of expertize needed to deliver the best.
-
5. Predictive Analytics Deliverables Overview
-
Define service offerings, service level expectations and point-of-contact process
-
Run Predictive Analytics Team as an Internal Consulting Team as a prerequisite to become a Strategic Business Unit (SBU)
-
Put clear processes in place to ensure how changes in the company’s strategy translates to changes in the Predictive Analytics’ strategy. Anticipate changes in the company’s strategy and be ready to respond rather than react.
-
Ensure that there is a process map within the Enterprise Analytics Team that shows which team is responsible for what, when do the Business Analytics collect the business requirements, when do the Technical Analytics convert them to Technical Requirements, Which Team get the requirements, the Predict Analytics? the Operations Analytics? the BI Reporting Analytics? The process map ensures that there is interworking relationship as part of the Enterprise Analytics Team as a whole.
-
Manage change and measure performance: The leadership should set clear expectation, implement change and measure overall performance
-
Strategic delivery congruence: The Predictive Analytics leadership has to ensure that there is a very good understanding of the congruence of the company’s strategic vision, mission, values and the Predictive Analytics organization’s strategic vision, mission, values and guiding principles. In addition, the Predictive Analytics Team has to have a very good understanding of:
-
Where is the company in term of maturity curve?
-
Where are the products and services in terms of their life cycles?
-
What are the different maturity curves for the different product lines and service lines? This has important not only for the deliverables of relevant predictive models but also scoring models that are put together to score accounts, customers in terms of churn, retention etc. The scoring of account performance in the early stage will probably not work very well if applied to old accounts.
-
Where is the industry in terms of maturity curve?
-
What are the dominant factors governing the tendencies within the industry?
-
What are the prevailing models in terms of:
-
Competitive Analytics
-
-
What are the prevailing models in terms of:
-
Business model - Example of the models include:
-
Manufacturing
-
Merchant
-
Commission
-
Advertiser
-
Brokerage
-
Franchise
-
Freemium
-
-
Revenue model - Example of the models include:
-
Production
-
Wholesale
-
E-commerce
-
Commission
-
Advertising
-
Licensing
-
Markup
-
Freemium
-
-
Marketing models - Example of the models include:
-
Customer experience
-
Customer engagement
-
Customer acquisition
-
Customer retention
-
Social media
-
CRM
-
Customer intelligence
-
Customer service
-
Digital marketing
-
Integrated marketing
-
Loyalty
-
Channel experience
-
Email marketing
-
-
Macro-environment based on:
-
Demographic changes?
-
Changing attitude and opinions
-
Size, structure, and distribution of the population
-
Changes in labor market
-
-
Economic changes?
-
Trends
-
Market cycles
-
Interest and exchange rates
-
Inflation
-
Consumer spending
-
Unemployment rate
-
-
Political changes?
-
Government policies
-
Political stability
-
Political ideologies
-
-
Legal changes?
-
Competition Law
-
Employment Law
-
Business Law
-
Health and Safety Law
-
-
Cultural changes?
-
Lifestyle changes
-
Consumer buying patterns
-
Religious factors
-
-
Technological changes?
-
Technological maturity
-
Technological solutions
-
-
Global changes?
-
Population
-
Birth, death and migration
-
GDP changes
-
Foreign investment
-
-
-
-
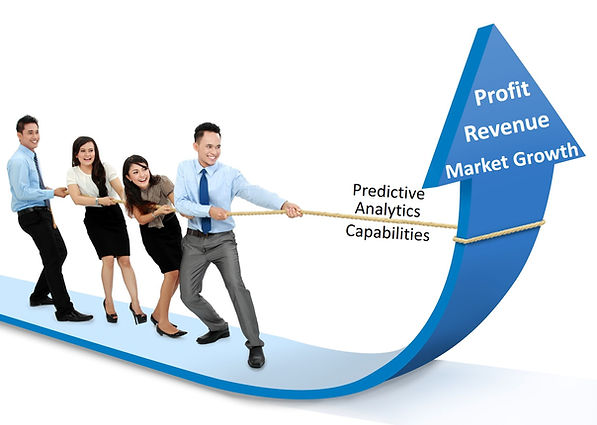
Strategic Responsibilities of the Analytics/ Predictive Analytics Leadership and Deliverables in terms of External Customer‐Facing Deliverables to support the Strategic Business Performance and goals
The external facing strategic deliverables for the Predictive Analytics Team and Leadership include:
-
Participate effectively in the pre-planning activities that can include workshops, workouts and brainstorming sessions
-
Have clear understanding of the stages in the planning process and the corresponding expectations of input from the predictive analytics team and leadership in each of the stages
-
Effectively ensure the alignment of the predictive analytics organization as strategically aligning to the overall company strategy.
-
Work with the Business Leadership to ensure that predictive analytics can best be used to:
-
Align how the company competes in a given industry - how the company creates value in an industry.
-
Implement the vision of what customers want and how the company can serve and deliver value to them
-
Combine specific activities and processes to enable the company to create unique value.
-
Align organizational activities so that all efforts are consistently reinforcing the potential advantage of the company
-
-
Align predictive analytics algorithms as intelligent enablers for corporate strategic plan.
-
Leverage different types of algorithmic techniques to support both the apparent and underlying factors in the company’s operations, revenue, marketing, and industry models with the dynamic complexities of macro-environment to provide alternative pathways and opportunities for business leaders
-
-
Enhance Strategic Planning through:
-
Predicting Strategic performance that:
-
Look at the whole gamut of business opportunities, including international expansion and mergers and acquisitions.
-
Define the organization’s values, expressed in financial and nonfinancial terms
-
Center on identifying and building or acquiring key resources and capabilities
-
Involve decisions about which industries the organization will compete in and how the businesses will be linked.
-
Determine how organizational resources will be allocated among the strategic businesses
-
Determine constraints on what the strategic businesses will and will not do
-
-
Predictive analytics should be able to include in the models and scores the factors that represent the threats and activities against which strategic performance are assessed and evaluated.
-
Strategic Financial Performance
-
Predicting earnings performance through:
-
Assessing whether its anticipated performance is in line with its established targets
-
Anticipating the amount of funding needed to achieve its forecasted sales growth
-
Estimating the effects of changes in assumptions of key numbers by performing sensitivity analysis (what-if analysis).
-
-
Business units’ performance
-
-
Strategic Process Performance – Predictive Analytics can be applied in areas where:
-
Interventions that are results of statistical designs
-
Changes in different types of cost as part of rework
-
Trajectory of business loss without the implementation of statistical control
-
-
Strategic Marketing Performance Modeling on:
-
Establishment of new business
-
Target market segments
-
Existing product and services performance
-
New product and services performance
-
Products and services – The markets where these products and services can have the highest returns
-
Who are the customers buying in the type of markets in terms of what to do to keep the customers coming back in terms:
-
Customer acquisition, churn, retention, customer lifetime value (CLV)
-
Measure of referral including Net Promoter Score (NPS) and satisfaction index
-
Profitability score of the customers
-
Customer complaints
-
Customer turnover rate
-
Customer buying behavior in terms of psychology, culture, subculture, family decision making, perception, attitudes etc.
-
-
-
Market segmentation
-
Product and services targeting and positioning
-
Cross-selling opportunities
-
Information search and decision Making
-
-
Returns on advertising campaigns
-
Service/product positioning
-
Cost and/or value leverage
-
-
Strategic Operations Performance Modeling on:
-
Strategic Performance Capacity
-
Performance capacity:
-
Capacity utilization
-
-
Inbound and outbound logistics
-
Inventory management
-
Supply chain management
-
IT performance
-
Procurement
-
Overall delivery system
-
Current and future capacity expansion
-
Process technologies and management including quality management
-
Facilities and location
-
Capacity management
-
Scheduling management
-
Continuous improvement
-
Changes to organization structure
-
Human resources management that include hiring, retaining, training, employee productivity, employee satisfaction and employee loyalty
-
Acquisition, adaptation and Implementation of new innovative technologies
-
Acquisition and implementation of improved production methods
-
Alignment in process design, re-design and improvement
-
Implementation of improved logistics
-
-
Enterprise Risk Management
-
Strategic risk management
-
Asset-Liabilities risk management
-
Securities allocation
-
Diversification
-
Total returns
-
Asset quality distribution
-
Market risk sensitivities
-
Interest rate sensitivity
-
Equity return sensitivity
-
Earnings at risk sensitivities
-
A/L Mismatch
-
Pre-hedged versus post-hedged value
-
-
Credit and/counterparty risk
-
Liquidity risk
-
Operational risk
-
Portfolio risk
-
Compliance risk
-
Marketing risks
-
Pricing risk
-
Balance sheet composition risk
-
Underwriting risk
-
Reserving risk
-
-
Capital Management
-
State of the company’s capital
-
Current changes in the capital position of the company
-
Actual versus expected
-
Capital injection
-
Drivers of the changes in the movement in the capital components
-
-
-
Adequacy of capital
-
Risk to capital
-
Capital at risk sensitivities
-
-
Emerging Risk Threat
-
Ability to respond to volatility in the market
-
Agility in being able to manage unexpected rapid change
-
Integrating the company strength while maximizing the opportunities to mitigate active and inactive threats
-

References
Banasiewicz, A. (2013). Marketing Database Analytics. NY: Routledge.
Barber, D. et al. (ed). (2001). Bayesian Time Series Models. Cambridge, UK: Cambridge University Press.
Capon, N. et al. (1992) Profiles of Product Innovators Among Large United-States Manufacturers. Management Science, 38 (2 ), 157-169
Carlin, Bradley, C. P. and Thomas A. L. (2009). Bayesian Methods for Data Analysis. Boca Raton, FL: Taylor & Francis Group, LLC
Christopher M. Bishop C.M. (2006). Pattern Recognition and Machine Learning. NY: Springer Science + Business Media, LLC
Chakrovarthy, B.S. (1982) Adaptation: A Promising Metaphor for Strategic Management. Academy of Management Review, 7, 35-44
Cohen and Levinthal (1990) Absorptive-Capacity - A New Perspective on Learning and Innovation. Administrative Science Quarterly 35(1), 128-152
Congdon, P. (2003) Applied Bayesian Modelling. New Jersey: John Wiley & Sons, Ltd.
Eisenhardt, K.M. and J.A. Martin (2000). Dynamic Capabilities: What Are They? Strategic Management Journal 21 (10/11): 1105-1121
Eisenhardt, K.M. and Graebner, (2007) Theory Building From Cases: Opportunities and Challenges. Academy of Management Journal, 50: 25- 32
Eugene, H. D. (2014). Bayesian Methods for Management and Business : Pragmatic Solutions for Real Problems. New Jersey: John Wiley & Sons, Ltd.
Farrar, C. R. and Worden, K. (2013). Structural Health Monitoring: A Machine Learning Perspective. West Sussex, England: John Wiley & Sons Ltd
Foss, L. et. al, (2009). The Role of Microfoundations in Explicating Dynamic Capabilities: A Case Study of Commercializing Discontinuous Innovation in the Norwegian Petroleum Sector
Freitag A. (2015). Applying Business Capabilities in a Corporate Buyer M&A Process. Fachmedien Wiesbaden: Springer
Geweke, J. (2005). Contemporary Bayesian Econometrics and Statistics. New Jersey: John Wiley & Sons, Inc.
Girdhar, P. et. al. (2004). Practical Machinery Vibration Analysis and Predictive Maintenance. Burlington, MA: An imprint of Elsevier, IDC Technologies.
Gopalakrishnan, S. et. al. (2011). Computational Techniques for Structural Health Monitoring. NY: Springer-Verlag
Greenberg, E. (2008). Introduction to Bayesian Econometrics. Cambridge, UK: Cambridge University Press
Griffith, D.A., and Harvey, M.G. (2001). A Resource Perspective of Global Dynamic Capabilities. Journal of International Business Studies, 32(3), 597–606.
Helfat, C. and Lieberman, M. (2002) The Birth of Capabilities: Market Entry and the Importance of Pre-History. Industrial and Corporate Change 11 (4): 725–760
Helfat, C. E., Finkelstein, S., Mitchell, W. Peteraf, M., Singh, H., Teece, D. and Winter, S. (eds.) Dynamic Capabilities: Understanding Strategic Change in Organizations. London: Blackwell.
Henderson, R. and Cockburn, I. (1994). Measuring Competence? Exploring Firm Effects in Pharmaceutical Research. Strategic Management Journal, 15(1): 63 - 84.
Jeff Gill, J. (2015). Bayesian Methods: A Social and Behavioral Sciences Approach, 3rd ed. Boca Raton, FL: Taylor & Francis Group, LLC
Jim Albert, J. (2009). Bayesian Computation with R. 2ed. NY: Springer Science + Business Media, LLC.
Jose J. Pazos Arias J. et. al. (2012). Recommender Systems for the Social Web. New York: Springer
Kéry, M. (2010). Introduction to WinBUGS for Ecologists : A Bayesian Approach to Regression, ANOVA,
Mixed Models and Related Analyses. Burlington, MA: Elsevier Inc.
Klugman, Stuart, K.A. (1992). Bayesian Statistics in Actuarial Science: With Emphasis on
Credibility. Massachusetts: Kluwer Academic Publishers
Koop, G. (2003). Bayesian Econometrics. West Sussex, England: John Wiley & Sons Ltd
Kuuluvainen, A. (2012). International Growth of a Finnish High-Tech SME: A Dynamic Capabilities Approach. Research in Economics and Business: Central and Eastern Europe, 4(2) 26-40.
Marin, J. and Christian, P. R. (2007). Bayesian Core: A Practical Approach to Computational Bayesian Statistics. NY: Springer Science + Business Media, LLC.
Miles and Snow, (1978) Organizational Strategy, Structure, and Process, West, New York, NY
Mitttal, A. and Kassim, A. (2007). Bayesian Network Technologies: Applications and Graphical Models
Hershey PA: IGI Publishing (an imprint of IGI Global)
Nicolas Werro, N. (2015). Fuzzy Classification of Online Customers. NY: Springer
Ntzoufras, I. (2009) Bayesian Modeling Using WinBUGS. New Jersey: John Wiley & Sons, Inc.
Parag Kulkarni, P. (2012). Reinforcement and Systemic Machine Learning for Decision Making. New Jersey: John Wiley & Sons, Inc.
Python Programming Books
R Statistical Programming Related
Rachev, S. T. et. al. (2008). Bayesian Methods in Finance. New Jersey: John Wiley & Sons, Inc
Ricci, F. et. al. (2011). Recommender Systems Handbook. NY: Springer
Rossi, P. E. et al. (2005). Bayesian Statistics and Marketing. NJ: John Wiley & Sons, Ltd.
Scutari, M and Denis, J. (2015). Bayesian Networks With Examples in R. Boca Raton, FL: Taylor & Francis Group, LLC
Schall. D. (2015). Social Network-Based Recommender Systems. NY: Springer
Teece, D.J., and Pisano, G. (1994). The Dynamic Capabilities of Firms: An Introduction. Industrial and Corporate Change, 3(3), 537–556
Teece, D.J., Pisano, G. and Shuen, A. (1997) Dynamic Capabilities and Strategic Management. Strategic Management Journal, 18, 509-533
Teece, D. J. (2007) Explicating dynamic capabilities: The Nature and Microfoundations of (Sustainable) Enterprise Performance. Strategic Management Journal, 28, 1319-1350.
Teece, D.J. (2009). Dynamic Capabilities and Strategic Management: Organizing for Innovation and Growth. New York: Oxford University Press.
Tomohiro., A. (2010) Bayesian Model Selection and Statistical Modeling. Boca Raton, FL: Taylor & Francis Group, LLC
Volberda, H. W. (1996). Toward the Flexible Form: How to Remain Vital in Hypercompetitive Environments. Organization Science, 7(4), 359-374.
Volberda, H. W. (1998). Building the Flexible Firm; How to Remain Competitive. Oxford: Oxford University Press.
West, M. and Harrison, J. (1997). Bayesian Forecasting and Dynamic Models. NY: Springer-Verlag.
Zahra, S. A. and George, G. (2002) Absorptive capacity: a review, reconceptualization and extension. Academy of Management Review, 27, 185-203