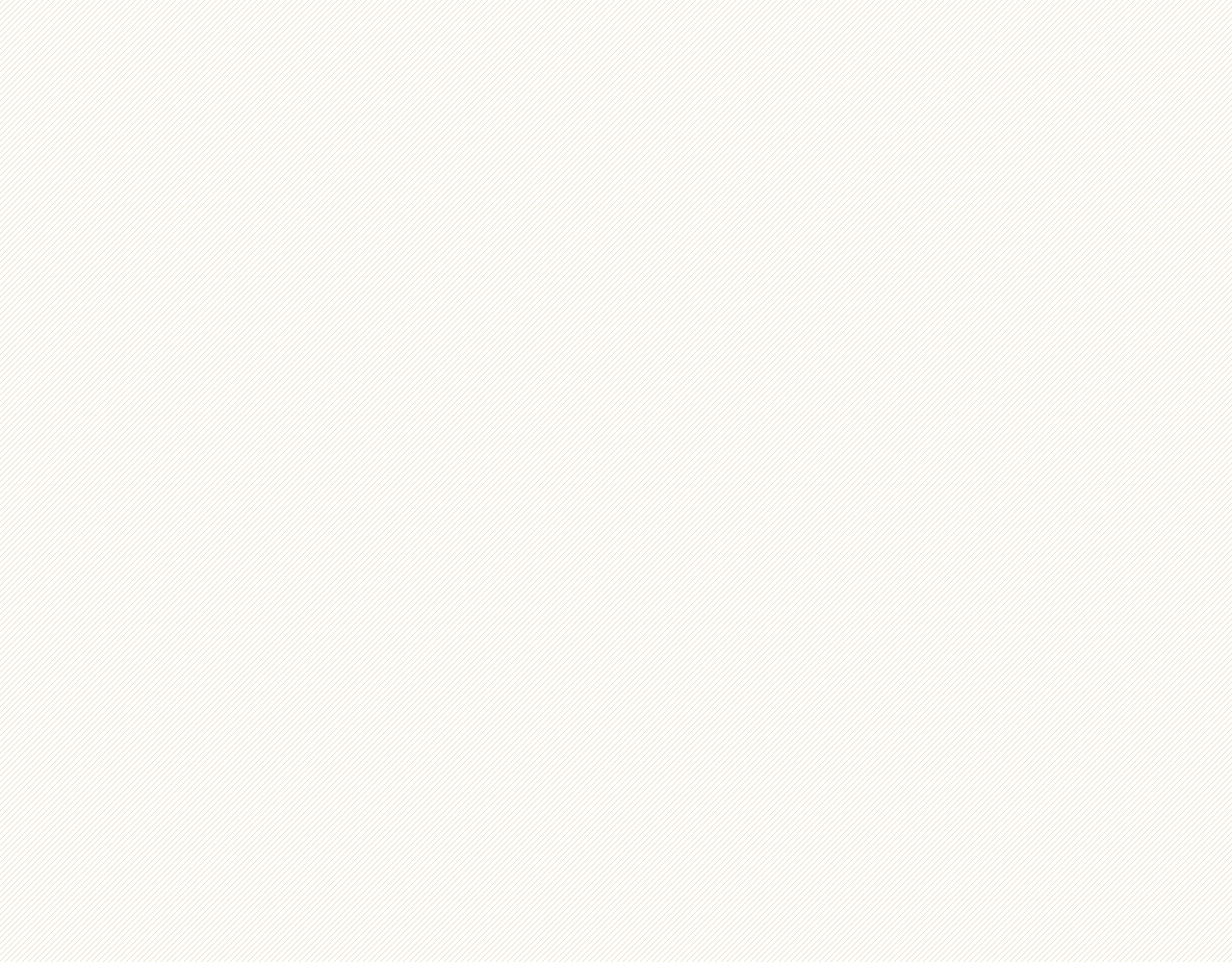






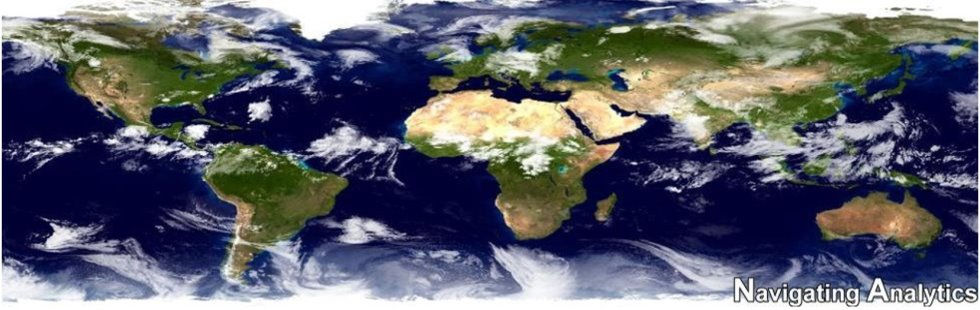
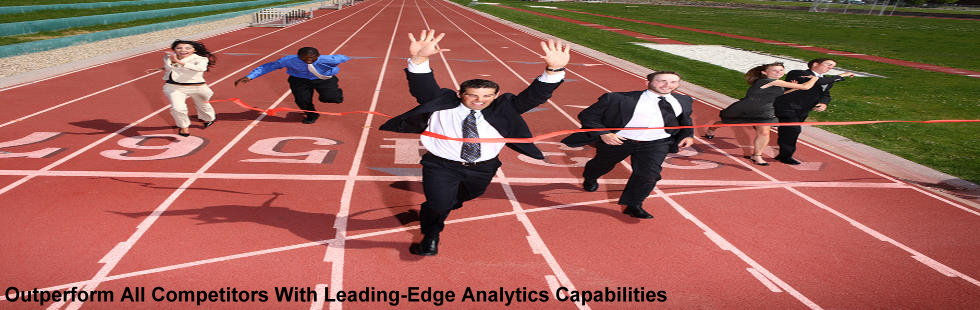
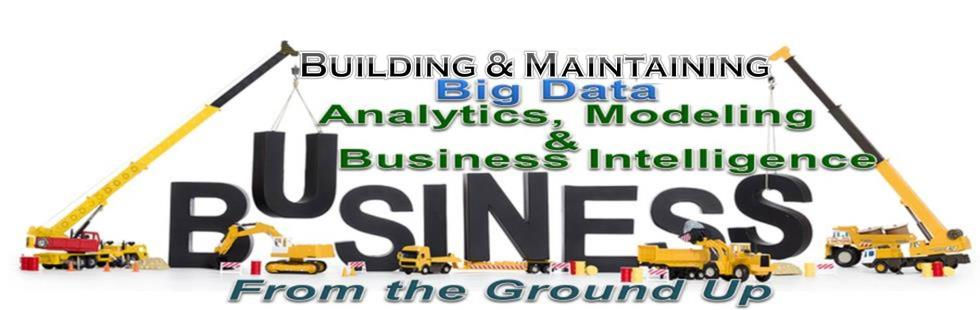
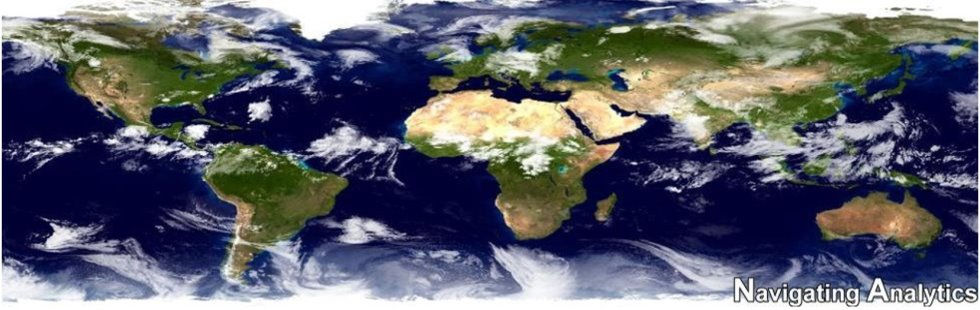
Navigating Analytics
Expertise in Building and Managing Teams, Structures and Processes for Data Management, Analytics, Modeling, Optimization, Business Intelligence Reporting Dashboards and Real-time Streaming from Cloud Technologies

Strategic Data: The Life Blood that Enables Efficiency, Growth, Revenue and Profitability
The executive leadership has to make decisions, communicate the decisions and ensure alignments and measurements across the organization in terms of efficiency, growth, revenue and profitability. Most of the time, the quality and strength of these decisions, communications, alignments and measurements come down to the quality, reliability and availability of the actionable data that the executive leaders are using.
A forward looking and shareholders’ value focused company ensures that its strategic planning is based on integrating the strength of its management with opportunities in marketing space along with effectiveness in its enterprise risk management and commitments to customer service so as to be able to drive the solidness of financial performance.
The basis of assessing the strength of the management including employees, the opportunities in the marketing space, the effectiveness of its enterprise risk management and commitments to the customers can mostly be measured by the use of data – whether qualitative or quantitative, whether structured, semi-structured or unstructured - In most cases, Small data combined with Big data.
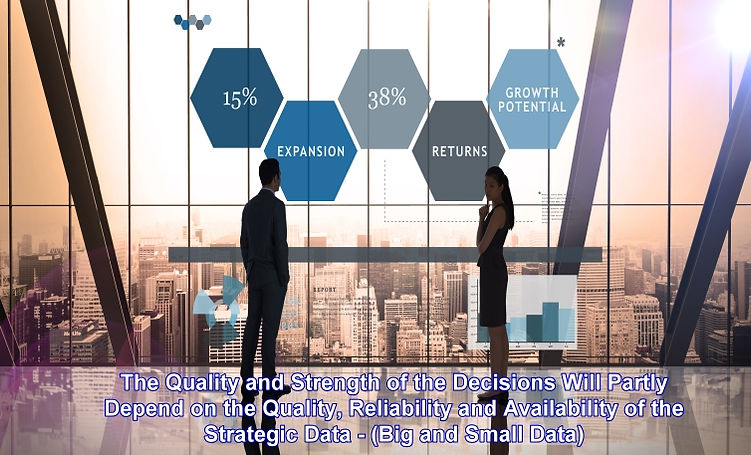
The depth of attention paid to the quality, reliability and availability of the data as input to the decision making process and the strategic planning process is also the reflection of the breadth and depth of results that the company is going to get from the decisions and the strategic plans. If there is shallowness in the depth paid to the quality, reliability and availability of the data, the decisions and strategic plans will in most cases be shallow, empty and will not go the distance.
Companies operate in competitive environments. While in some industries, the competition is moderate and manageable. In many industries, the competition is intense, fierce and unpredictable. For the companies to effectively and efficiently maneuver at strategic and tactical levels, they have to focus on how best they can use their data as strategic capability.
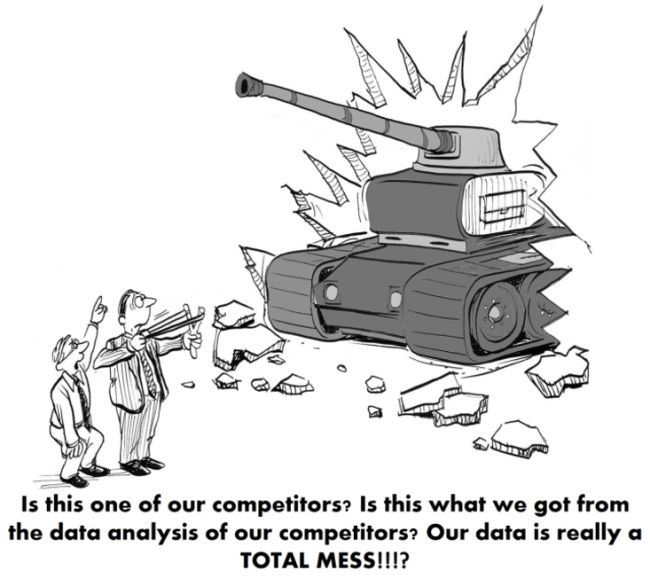
Data as data or raw information in itself does not make it strategic. What makes data strategic is positioning of the data based on the expected value-added that the data can provide to support and drive business results either in short, medium or long term in relationship with the direction that the company wants to take as part of its vision, mission and strategic plan. In additional, data is strategic based on being able to use the data to generate competitive and financial advantages that have short and long term profound effects in how the company breaks out of the pack and sustain the increasing values that are generated for all the stakeholders and the shareholders.
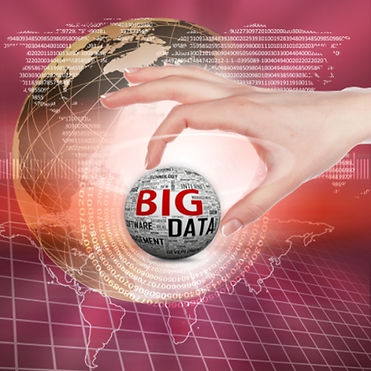
Whether the company is concerned with performance to maintain its share of the current market or get into a new promising market or is concerned with keeping share of wallet in terms of customer churn, customer buying habits and the new regulation coming into play across the board, the company needs data. One of the symptoms of many of the struggling companies is that they treat their relationship with data as a “reacting” or “emergence” situation. The request for data from the Data Management Team is like calling 911 for help. Many companies spend tremendous amount of time and focus on data request intake process. Even when the data request intake process is design, redesign or/and improved, there are still problems in getting the data that is needed because the relevance of the data to the problems or issues that the company is trying to solve is also important just like the request intake process. Many at time, there are data requests without a clear understanding of the data requirements. Before even making requests that have clarity with the data requirements, there are no clarity with the metrics requirements or/and the reporting requirements.
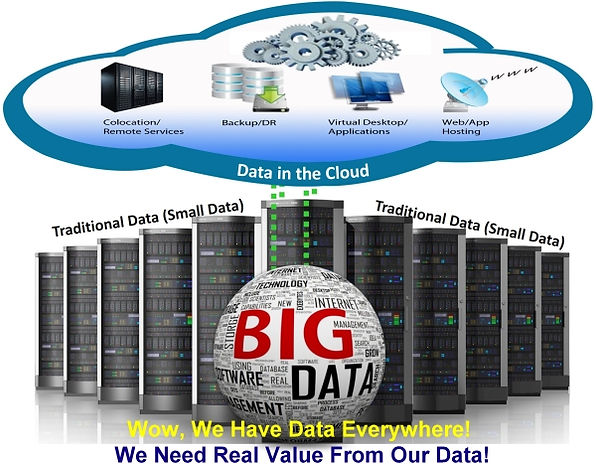
Driving for strategic data management is the pre-cursor for generating a strategic plan and tactical action plans that can mostly stand the test of time and survive the typical onslaughts from competitive environment. Navigatinganalytics.com provides information on how best to get the most out of strategic data through:
-
Driving Business Performance
-
Strategic Customization of the Suggestions in Strategic Data to Fit and Benefit Your Business
-
Strategic Data Alignment
-
Two Types of Value Propositions
-
Building Blocks for the First Value Proposition
-
Strategic Data Capabilities: Scanning Gap Assessment of the Current Data Capabilities
-
Strategic Data Capabilities: Assessment of Measurable Benefits
-
Strategic Data Capabilities: Assessment of Different Options
-
Strategic Data Context
-
Implementation or/and Project Readiness Before the Building Out of the New Capabilities
-
Building Out the Execution Plan for the New Capabilities: Data Organization
-
Defines and Develops Data Governance Structure
-
Defines, Designs and Build-out Strategic Data Organization Model
-
Data Operating Model
-
-
-
Post Implementation: Managing Blocks for the Second Value Proposition
-
Drives Predictive Analytics and Performance Metrics for Profitability: Use advanced statistical analysis and modelling including predictive statistical methods to identify the factors to ensure business-line profitability
-
Leveraging Analytics Best Practices to Measure the Impact on Business Results
-
Leverages the enterprise data through the application of sophisticated analytic methods to provide insights into Strategic Planning
-
Performance Management, Sensors and Timely Corrective Actionable Plan
-
-
Analyzes the enterprise data to understand the patterns, trend and “what next”. Creates environment that allows for objective identification and measurement of drivers that can be influenced if there is the need to change the current course of action(s) through the Command Center:
-
Command Center Deliverables
-
-
Creates structures and processes that allow for objective identification and measurement of drivers that can be influenced if there is the need to change the current course of action(s):
-
Operational Reporting, Dashboards and Scorecards on the performance of the organization
-
Enterprise Risk Management (ERM) Deliverables: Leverage the enterprise data through the application of sophisticated quantitative analytic methods to provide insights that management can use to proactively manage critical business risk issues.
-
-
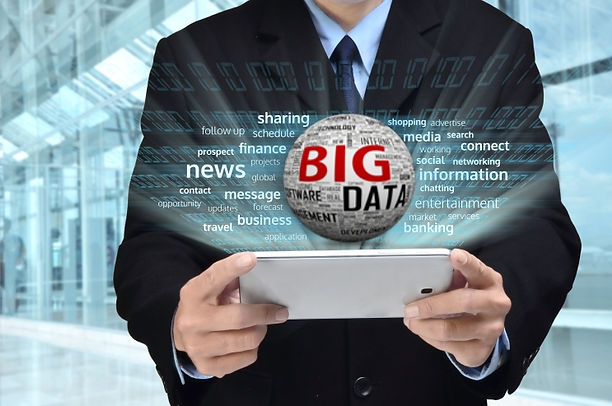
Driving Business Performance:
Strategic data management services are needed to ensure that the company can use its local and global data to drive Business Performance that include:
-
Strategic Financial Performance
-
Earnings performance
-
Balance sheet performance
-
-
Business units performance
-
Investment performance
-
-
Strategic Process Performance
-
Process performance
-
-
Strategic Marketing Performance
-
Establishment of new business
-
Target market segments
-
Existing product and services performance
-
New product and services performance
-
Products and services – The markets where these products and services can have the highest returns
-
Who are the customers buying in the type of markets in terms of what to do to keep the customers coming back in terms:
-
Customer acquisition, churn, retention, customer lifetime value (CLV)
-
Measure of referral including Net Promoter Score (NPS) and satisfaction index
-
Profitability score of the customers
-
Customer complaints
-
Customer turnover rate
-
Customer buying behavior in terms of psychology, culture, subculture, family decision making, perception, attitudes etc.
-
-
-
Market segmentation
-
Product and services targeting and positioning
-
Cross-selling opportunities
-
Information search and decision Making
-
-
Returns on advertising campaigns
-
Service/product positioning
-
Cost and/or value leverage
-
-
Strategic Operations Performance
-
Inbound and outbound logistics
-
Inventory management
-
Material requirement planning
-
Enterprise resource planning
-
Just-in-time management
-
-
Supply chain management
-
IT performance
-
Procurement
-
Overall delivery system
-
Current and future capacity expansion
-
Process technologies and management including quality management
-
Facilities and location
-
Capacity management
-
Scheduling management
-
Continuous improvement
-
-
Changes to organization structure
-
Human resources management that include hiring, retaining, training, employee productivity, employee satisfaction and employee loyalty
-
Acquisition, adaptation and Implementation of new innovative technologies
-
Acquisition and implementation of improved production methods
-
Alignment in process design, re-design and improvement
-
Implementation of improved logistics
-
Enterprise Risk Management
-
Strategic risk management
-
Asset-Liabilities risk management
-
Securities allocation
-
Diversification
-
Total returns
-
Asset quality distribution
-
Market risk sensitivities
-
Interest rate sensitivity
-
Equity return sensitivity
-
Earnings at risk sensitivities
-
A/L Mismatch
-
Pre-hedged versus post-hedged value
-
-
Credit and/counterparty risk
-
Liquidity risk
-
Operational risk
-
Portfolio risk
-
Compliance risk
-
Marketing risks
-
Pricing risk
-
Balance sheet composition risk
-
Underwriting risk
-
Reserving risk
-
-
Capital Management
-
State of the company’s capital
-
Current changes in the capital position of the company
-
Actual versus expected
-
Capital injection
-
Drivers of the changes in the movement in the capital components
-
-
-
Adequacy of capital
-
Risk to capital
-
Capital at risk sensitivities
-
-
Emerging Risk Threat
-
Ability to respond to volatility in the market
-
Agility in being able to manage unexpected rapid change
-
Integrating the company strength while maximizing the opportunities to mitigate active and inactive threats
-

Strategic Customization of the Suggestions in Strategic Data to Fit and Benefit Your Business
Before leveraging some of the suggestions below, there is the need to ask the questions below so that many of the suggestions below can be customized to benefit your business. Many of the suggestions will need to be customized given the nature of business and the difference in the maturity curves. Some of these questions include:
1. What type of business model do you have?
Example of the models include:
-
Manufacturing
-
Merchant
-
Commission
-
Advertiser
-
Brokerage
-
Franchise
-
Freemium
2. What is your revenue model?
Example of the models include:
-
Production
-
Wholesale
-
E-commerce
-
Commission
-
Advertising
-
Licensing
-
Markup
-
Freemium
3. What are the levels of maturity in the marketing models in your company?
Example of the models include:
-
Customer experience
-
Customer engagement
-
Customer acquisition
-
Customer retention
-
Social media
-
CRM
-
Customer intelligence
-
Customer service
-
Digital marketing
-
Integrated marketing
-
Loyalty
-
Channel experience
-
Email marketing
4. What are the demands of your stakeholders in the macro-environment based on:
-
Demographic changes?
-
Changing attitude and opinions
-
Size, structure, and distribution of the population
-
Changes in labor market
-
-
Economic changes?
-
Trends
-
Market cycles
-
Interest and exchange rates
-
Inflation
-
Consumer spending
-
Unemployment rate
-
-
Political changes?
-
Government policies
-
Political stability
-
Political ideologies
-
-
Legal changes?
-
Competition Law
-
Employment Law
-
Business Law
-
Health and Safety Law
-
-
Cultural changes?
-
Lifestyle changes
-
Consumer buying patterns
-
Religious factors
-
-
Technological changes?
-
Technological maturity
-
Technological solutions
-
-
Global changes?
-
Population
-
Birth, death and migration
-
GDP changes
-
Foreign investment
-
-
Navinganalytics.com views strategic data alignment as sets of well thought-out integrated and related activities that leaders have to take with the explicit and implicit intentions of leveraging internal, external, big and small data to enhance the capabilities of the given company to deliver superior performance to the employees and shareholders relative to its competitors.
The success of strategic data alignment depend on being able to create a data strategy that combines the value proposition which encourage the business to want to invest in the data as part of the company’s capabilities and the revenue enhancing proposition that the company believe that data capabilities will provide the edge in the competitive environment.
Strategic Data Alignment
With that in mind, there are two value propositions for the data strategists in any company to work with.
Two Types of Value Propositions
-
The first type of value proposition is that the company has to accept the principles that in order to be able to collect, know, standardize, access, use, derive values and secure its data, it has to invest in the data as part of the company’s capabilities.
-
The second type of value proposition is that once these capabilities are planned, built or acquired and implemented, these capabilities have to be leveraged correctly to generate return on investment of the capital invested in data, analytics and business intelligence tools, technologies, infrastructures and manpower. The capabilities will have to be designed to handle large volumes of data so as to derive operational intelligence. Also, this value proposition implies explicit and implicit enhancement of functional benefits, productivity, revenue and profitability otherwise, the investments are waste of scarce resources that could have been deployed somewhere else in the company.

Building Blocks for the First Value Proposition include:
Business Case – Quantification of the Value Proposition
In many cases, the underlying justifications for the business case of the first type of value proposition are derived from conducting Voice of Customers (Executive, employees and other key stakeholders) Assessments that focus on understanding the type of business, the needs of the business, the current capabilities of the business and the expected capabilities that the business is looking to add, replace or/and modify.
There are different analytics methods to help get to a less subjective VOC assessment. These include the use of cohort analysis, segmentation analysis and multivariate analysis that include factor analysis, cluster analysis, neural networks, discriminant analysis and simultaneous equation modeling. Also in addition, there are six sigma and lean approaches that include affinity diagram, Kano Model, Critical to Quality (CTQ), Quality Function Deployment, TRIZ, Design of Experiment and statistical analysis.
The logics of the post Voice of the Customer assessment can be divided into 3 groups:
1. Strategic Data Capabilities: Scanning Gap Assessment of the Current Data Capabilities:
Assessment of the different dimensions in current data capabilities spectrum, the missing gap and what will be needed in the future data capabilities along with the options that can best be used to build the needed capabilities that support both small and big data
(a). The assessment of current and future data capabilities include but not limited to:
-
Data Strategy
-
Data Governance Model
-
Data Organization Model
-
People and Organization
-
-
Data Operating Model
-
Data Operations Management
-
Processes
-
Technology Infrastructure
-
Metrics
-
-
Data Architecture Management
-
Data Infrastructure Management
-
Data Integration Platform
-
Scalability
-
Adaptive to different data sources
-
Capability for parallel data streaming
-
Multithreading capacity
-
Generation and integration of metadata
-
-
Data Warehousing and Database Intelligence Management System
-
Data Conversion and Extraction
-
Data Retention and Archiving
-
Meta Data Management
-
Data Development
-
Data Security Management
-
Data Quality Management
-
Master Data Management
-
Reference Data Management
-
Document and Content Management
-
Digital Interfaces
-
Big Data Management including Analytics
(b). The assessment of current and future data related processes that include but not limited to:
-
Data Policies
-
Data Ownership
-
Data Standards
-
Data Stewardship
-
Data Dictionary
-
Data Security and Privacy
-
Data Lineage
(c). The assessment of current and future data related basic technologies that include but not limited to:
-
Enterprise Data Acquisition Domain including ingestion, Open Source Component Management and Staging
-
Enterprise Data Marshalling Domain
-
Enterprise Data Processing Domain including Data Protocols, Data Stream Capabilities, Data Accelerators and Complex Event Processing
-
Master Data Management (MDM)
-
Enterprise Data Integration
-
Pre-preprocessing
-
Data Profiling
-
Staging Area
-
Data Transformation
-
Metadata Management
-
Data Modeling
-
Data Quality
-
Data Cleaning
-
Data ETL
-
-
Enterprise Data Hubs and Architecture including for example:
-
Data Centers and Data Warehouse
-
Cloud Computing – Infrastructure, platform and applications
-
(d). The assessment of current and future data related organization that include but not limited to:
-
Mission, Vision, and Objectives
-
Organization Model
-
Current
-
Transactional
-
Future
-
-
Roles, Responsibilities and Skills
-
Training Requirements
-
Organization Model Deployment or Redeployment.
This involves:
2. Strategic Data Capabilities: Assessment of Measurable Benefits
Identification of the needed or future data capabilities based on the gap analysis in terms of measurable benefits that can be realized.
Some of the measurable benefits that can be realized in developing data capabilities include:
(a). Alignment with the Business Strategy to:
-
Deliver value-added data when, where and how it is needed for effective decision making on growth of market share, generate increase revenue and improve on sustainable profitability
-
Enhance the enabling capacity or serve as enabler in increasing operational sales, increasing efficiency, reduce waste, mitigate fraud and improve effectiveness of operational processes and reducing cost through increase in efficiency
-
Provide complete customers’ information for the business to adjust its strategy as needed to make the most of the relationship with the customers in terms of product/service satisfaction
-
Reduce the time to market and be prepared to make agile changes whenever needed to adjust the projects, products, services and processes to align with the expectation of the customers.
(b). Operational Excellence that:
-
Ensure accuracy of data delivered to the business, technical, process and external (for example, Customers, Investors, Rating Agencies etc.) stakeholders. A conscious drive for “one single source of truth”
-
Enforce data governance to reduce the proliferation of metrics, drive operational security and provide accountabilities needed to ensure the consistency of defined processes, policies and procedures
-
Monitor data quality to quickly react to, and resolve accuracy issues
-
Standardize data quality to drive measurement consistency
-
Provide consistent methods and tools for data process modeling across the enterprise
-
Leverage the best applicable tools, technologies and processes to reduce costs and develop resource expertise in data management
-
Lead the automation of data processes to enable active performance measurement and monitoring
-
Enhance operational efficiency to:
-
Drive for consistency in standards, processes and rules in how the data is collected, defined, stored, retained, used and/or disposed and/or destroyed.
-
Assist data process owners in optimizing efficiency, increasing productivity, and reducing costs in the ways that data is collected, defined, stored, retained, used and/or disposed and/or destroyed.
-
(c). Data Process Mapping Designed or Improved to ensure :
-
Data Usability
-
Data Accuracy
-
Data Security
-
Data Consistency
-
Data Transparency
-
Corporate and User Trust in the Data
-
Data Availability
3. Strategic Data Capabilities: Assessment of Different Options
-
Cost-Benefit Analysis
-
Compatibility with existing capabilities
-
Features and flexibilities of the capabilities
-
Stability of the capabilities
-
Opportunities for future integration, development, improvement and maintenance
-
Expected values to be derived from investment analysis:
-
Increase in overall current performance to include:
-
Drive for consistency in standards, processes and rules in how the data is collected, defined, stored, retained, used and/or disposed and/or destroyed.
-
Increase in sales
-
Increase in revenue
-
Efficiency
-
Savings
-
Growth – Market share, Sales, Revenue, Profit
-
Reduced cost
-
Ensure regulatory compliance
-
Mitigate risks
-
Strategic, process and technical alignment with the current capabilities
-
Implementation cost in terms of the changing of priorities for the current staff, the use of consulting resources and capacity allocation
-
-
Expected use now and in the future
-
Long term support structure and process of the capabilities
(a). Assessment of options that can best be used to build the needed capabilities. The assessment include but not limited to:
(b). Assessment of soft options that can best be used to ensure the roll-out, adoption and sustenance of the needed capabilities.
-
Gap assessment of skillset inventories: current vs transitional vs. final/optimized skillsets
-
Culture
-
Communication
-
Ease of training and usage
-
Change management
-
Execution of portfolio, program and project management
-
Process Management that:
-
Drive for consistency in standards, processes and rules in how the data is collected, defined, stored, retained, used and/or disposed and/or destroyed.
-
Ensure the alignment of processes- Designing and building the new Process (Design for Six Sigma -DFSS), Process Improvement (DMAIC) and Lean Sigma
-
Emphasize Process mapping
-
Clearly layout the standard operating procedures (SOP)
-
-
Enterprise risk management including identification, measurement, control, monitoring and reporting capabilities
-
Performance management including measuring, monitoring and reporting capabilities
The assessment includes but not limited to:
(c). Assessment of Alternative Options to include:
-
Outsourcing which covers -
-
Risk
-
External factors to include existing and/or future regulations
-
Type of Service models
-
Deployment models
-
Service Level Agreements
-
Strategic and operations measurements
-
(d). Justification and Recommendation of options to be used to build the new capabilities
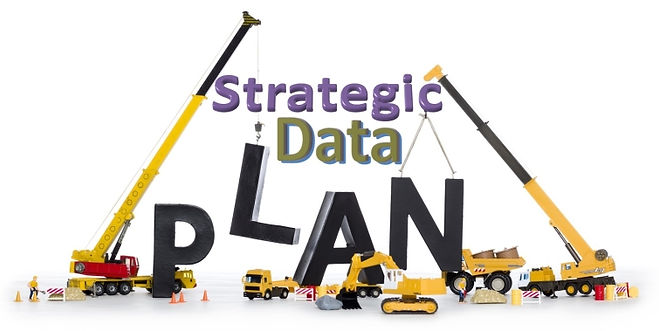
Strategic Data Context:
This involves the detailed description of critical business, technical and process deliverables that have to be developed based on the gap analysis or what can be considered the precursor to the successful implementation of the data strategy.
The strategic data context includes:
-
Detailed strategic data plan that clearly links data management best practices initiatives to the company strategic goals, priorities and fulfillments in the context of developing or building the needed capabilities as identified above. The strategic context includes robust scenario themes that lay out the different choices along with the greatest benefits and impacts for the company
-
The detailed strategic data plan is validated and refined based on the discussions and consensus with the Board, Senior Leadership Team (SLT) and other Stakeholders with the primary intention on achieving the greatest benefits and impacts from data collection, storage, ownership, usage and maintenance
-
Assessing and finalizing the company's data management readiness capabilities at the Corporate and Business Unit Levels through gap assessment to determine:
-
The degree to which existing data solutions can be integrated into Data, Analytics, Modeling and Business Intelligence (BI)/Reporting requirements
-
The level of internal capabilities of the existing data management staff to meet high level requirements defined in implementation plan
-

Implementation or/and Project Readiness Before the Building Out of the New Capabilities:
I.Business Readiness:
-
Identify key business uncertainties surrounding strategic data implementation
-
Assess and prioritize stakeholders' expectations based on preparedness and capabilities assessment
-
Assessment of the current environment for changes
-
Assessment of the costs and benefits of implementation
-
Validation plan for actual funding commitments
-
Assess timeline of important external and internal dependencies and the consequences on major execution of major projects
-
Coordinate the project schedules, obtaining concurrence and commitments from all parties involved
-
Develop core Strategic Data Team preparedness and capabilities. Deploy the capabilities to implement the project plans
-
Assess the needs for training (for the Strategic Data team and project team) if needed
II.Operational Readiness:
-
Determine the key stakeholders' involvement. Assess where the stakeholders are in terms of level of commitments. Get a sign-off by all or most members of the SLT on the master project plan, business units' and functional areas project charters
-
Focus on project management activities to ensure that project objectives are met within the parameters of approved scope, budget, quality and timeline
-
Assess economic, technical and company feasibilities that are needed for projects initiations
-
Identify key process uncertainties
-
Identify and evaluate Work Breakdown Structure (WBS) elements using the project risk areas to determine risk events with assignment of probability/likelihood and consequence/impact of each risk event to establish a risk rating
-
Map out projects to be executed on several key levels (Corporate, business units and functional levels) within the master project plan with a consistent focus on cost, schedule, quality, risk and resources
-
Determine the phases of strategic data implementation - Divide the strategic data implementation projects into several major phases to be done serially and within each phase, set to accomplish a variety of individual tasks simultaneously. Estimate duration of the each phase of the projects
-
Identify the key principles and dependencies that can influence the implementation roadmap
-
Develop and/or adopt operation support processes
-
Integrate strategic data requirements with operational support structures and processes
-
Identify all resources that will be required during each phase of the implementation and provide an estimate of efforts for these resources
-
Identify the pre- and post- implementation training that may be recommended for strategic data solutions. Describe the purpose and audience for each training requirement
-
Describe the preferred approach to plan, manage, deliver and/or coordinate training required
-
Determine which data structures and processes will require to be changed directly in order to achieve the strategic data implementation objectives or to support the required changes in other processes that lie within the Master Project Plan
-
Determine the nature of changes that will have to be undertaken, when, how, by who, in what order and with what possible constraints
-
Finalize requirements and scope of implementation
-
Develop and execute communication plan. Schedule update with all stakeholders
-
Adopt target measurement and regular reporting schedules
III.Technical Readiness:
-
Assess and determine the Strategic Data Technical Requirements. Share the Technical Requirements with appropriate teams or/and individual and have a consensus on a high level overview of the technical design requirements
-
Identify key technical uncertainties
-
Describe the infrastructural architecture of the proposed solutions. Include infrastructure architecture diagrams, assumptions for the infrastructure architecture, preferred major application components/modules and model deployment
-
Develop a detailed Architecture Design for the integration of Strategic Data Technical Requirements with the technological capabilities of the company. Assess the need for upgrading of appropriate risk facilities
-
Work with the IT Leadership Team to define and establish required environments for implementation areas that will require development, test, production and training among others
-
Put together an Enterprise Architecture strategies and tradeoffs for implementing robust, secure, high-performance, and high-availability solutions that are relevant to the successful implementation of Strategic Data objectives
-
Work with the IT Leadership Team to test and validate the technical environment
-
Assess and make recommendations that will be needed for the development of the Strategic Data dashboard
-
Assess the sources and stability of data quality that will be needed for the Strategic Data processes that include identification, measurement, control and reporting
IV.Change Management Readiness:
-
Work with different corporate and business unit leaders at various levels on the strategic data processes as they relate to the consequences in how the deliverables of the stakeholders will change.
-
Collaborate with the necessary stakeholders in putting together Enterprise Architecture strategies and tradeoffs for implementing robust, secure, high-performance, and high-availability solutions that are relevant to the successful implementation of Strategic Data solution. These covered the data, metrics and reporting requirements
-
Treat operating and culture change as proactive processes and structured approaches to address the people (leadership vision, employees’ engagement and stakeholders’ concerns) and organizational risks inherent in any change effort, to optimize business benefits realization/ROI, and to sustain long-term performance within a constantly changing environment
-
When necessary, influence without authority to successfully secure the sponsorship and support of various business and technical partners to achieve project goals and objectives
-
Demonstrate flexible approaches and resilience to setbacks and ability to drive operating and culture change
V.Internal Project Management Office (PMO) – Strategic Data Project Portfolio Management Readiness:
-
Focus on strategic data project management activities to ensure that data objectives are met within the parameters of approved scope, budget, quality and timeline
-
Map out strategic data projects to be executed on several key levels (Corporate, business units and functional levels) within the master project plan with a consistent focus on cost, schedule, quality, risk and resources
-
Determine the phases of strategic data implementation - Divide the strategic data implementation projects into several major phases to be done serially and within each phase, set to accomplish a variety of individual tasks simultaneously. Estimate duration of the each phases of the projects
-
Identify the key principles and dependencies that can influence the implementation roadmap
-
Develop and/or adopt operation support processes for the effective implementation of strategic data across the company
Define and Develop Data Governance Structure
The Data Governance Structure outlines the roles and responsibilities of those within the Data Management space to manage and govern the integrity of the data provided. Additionally, the structure identifies who will be responsible for monitoring and maintaining the quality of the data used to generate the performance metrics for the businesses. The Structure includes:
-
Roles and responsibilities
-
Accountabilities
-
Communication and awareness
-
Policy Management
-
Resources Allocation
-
Escalation process
-
Modification of governance sub levels for example in the business units
-
Enforcement of rules that include the components of enterprise risk management
-
Critical data elements
-
Data Stewardship
Building Out the Execution Plan for the New Data Capabilities:
Data Governance

Other elements of the Governance include:
Data Governance Principles
Principles are standardized in terms of defining the data quality goals to meet the needs of the user community. These include:
-
Governance Activity Matrix
-
Transparency
-
Auditability
-
Accountability
-
Stewardship
-
Checks-and-Balances
-
Standardization
-
Respect for context
-
Change Management
-
Enforcement
-
Point of contact for data processes
-
Rules of engagement with data customers/users
-
Data delivery methods
Data Processes and Procedures
Processes and Procedures define the rules of engagement between those representing the data organization and relevant parties including those from the business units. These include:
-
Guiding documentations on engagement with data customers/users
-
Business and Technical requirements documentation
-
Productivity Workflow Optimization Processes
-
Processes for data requirements
-
Processes of Governance Activity Matrix
-
Standardization of procedures
-
Documentation on central repository of data elements and data metrics
-
Data definitions
-
Metadata
Data Quality Tracking
Refers to the design, development, and implementation of the reports and scorecards used to track the quality of the data performance metrics as well as track the quality of the internal Operating Model processes within the data team. These include:
-
Standardization of metrics
-
Metrics monitoring
-
Scorecards
-
Design effectiveness
-
Operating effectiveness
-
Quality of deliverables
Data Architecture
Provides guidelines and direction as to the recommended path of retrieving the information needed to support the performance metrics as well as the implementation of the recommended data control and monitoring processes to enforce the data quality goals of each performance metric. These include:
-
Information Architecture
-
Data Accelerators
-
Applications
-
Connectors
-
-
Open Source Component Management
-
Technology Architecture
-
Technology Requirements
-
Data Collection - Data collected from multiple sources
-
Storage
-
Speed of delivery
-
Accessibility
-
-
-
Data Solutions Architecture
-
Processing
-
Efficiently designed
-
Self-healing and self-optimizing
-
-
Security protocol and control
-
-
Business Data Architecture
-
Big Data Enterprise Engines
-
Data service-oriented architecture
-
Data process architecture
Define, Design and Build-out Strategic Data Organization Model
The Strategic Data Organization Model has the responsibility to:
-
Establish Strategic Data Management Organization that promote and support data management activities
-
Provide the governance, standards, skills, and tools necessary to ensure an effective data management solutions
-
Drive the development and execution of data management strategy with strategic, business, process and technical deliverables that can sustain critical business requirements that are needed to support the overall business strategic imperatives. The strategic, business, process and technical deliverables from data management strategy must be tied to the strategic imperatives of the company
-
Determine the data management solutions and creates the standards, policies, procedures, and governance processes required to support enhanced and effective data management capabilities
-
Formalize the purpose, role, services, and structure of the data development by design
-
Assist Operational Business Leaders in developing tactical and strategic procedures to support the business’s goals (financial, quality, production, operational efficiency, etc)
There are prominent roles and teams within the Strategic Data Organization that are required as basic requirements for efficient and effective functioning. These include:
Chief Data Officer (CDO)
-
The CDO must understand how to run data management as if he/she is running the entire company. This mentality or understanding must be matched with the knowledge and understanding that running a bad data management strategy is the precursor to running the company out of business.
-
There is the expectation then that CDO must establish long-term strategy, vision, and goals for the entire Strategic Data Team which ensures that the organization has access to quality and reliable data. The strategy should be such that the structures, processes, technologies and people within the team can provide the pathway in data management that drives efficiency and effectiveness that allow the business to achieve corporate goals and objectives.
-
CDO serves as an expert on all aspects of the Data processes and management. Provides the internal facilitation for the governance committees through the business unit levels up to the corporate level.
-
CDO defines and drives adoption of best practices in the Data Management space.
-
CDO has the accountability and ownership of operational data successes and failures as they relate to the company deliverables.
-
CDO collaborates with Senior Leadership to develop and implement data infrastructure of systems, structures, processes, and personnel designed to successfully implement data management deliverables.
-
CDO is responsible for overall management of the fundamentals in data system architecture, data processes, database management, data infrastructure scalability, data security and digital data rights management.
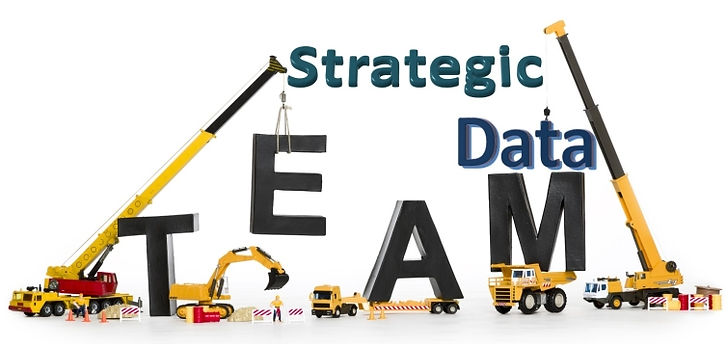
Advanced Data Stewardship Services Team
Data Stewardship Services Team maintains the company’s data assets to improve data quality, reusability, and accessibility and is organized across multiple business units. This involved working closely with the Data Quality Analysts and Security administrators from IT to define the data quality standards and security measures. The Data Stewardship team is responsible for the collection and administration of metadata, data profiling, data quality, data integrity, and benchmarking the user environment against the data governance standards. This team maintains the data assets to improve accessibility. Data Stewards understand the full front to back flow of the data set, not only from systems and feeds perspectives, but from sources, users and usability perspectives. Data Stewards are responsible for developing the processes, policies, and metrics to improve and maintain the quality of data in their domains. This team supports the Data Architect team by offering data modeling, database administration, vendor application administration, data profiling and data testing parameters and services.
The Data Stewardship is organized across multiple business units with a Principal Data Stewards at Corporate level to facilitate the coordination of all the Business Units.
Data Architecture Team
The Data Architect Team manages, builds and maintains the data architecture to absorb and scale data collection from multiple inputs and multiple market verticals. The team provides the guidelines and direction as to the recommended path of retrieving the information needed to support the performance metrics as well as the implementation of the recommended data control and monitoring processes to enforce the data quality goals of each performance metric. In working with the Data Stewardship Team, this team also ensures the publication and the integrity of the Data Reference Model and the Data Programs.
Data Quality Analytics Team
Data Quality Analytics Team defines the data quality standards and definitions required to meet the metric requirements of the user community. This includes initial assessments of the quality of the data from the various systems of record relevant to the requested metrics as well as the definition of the business and/or technical rules to meet the data quality targets expected by the user community. Moreover, Data Quality Analytics are responsible for defining the data quality controls required to support the integrity of the performance metrics managed by the Analytics Team working with either the Data Stewards or those within the Data Management Team.
Data Quality Control Team
Data Quality Controls Team has the responsibility to continual monitor the integrity of the data metrics over time. The quality control includes those embedded within the ETL processes which track use of the business and technical rules, reconciliation logic to verify that the information captured for each metric is consistent with other information within the company, as well as referential integrity checks.
Data Standardization Team
This team handles the creation and maintenance of standardized definitions for performance metrics, data elements, attributes, and schemas. Information such as naming standards, data classifications, business rules, data models, data dictionary information, and data format standards which are shared with users within the company so as to be able to leverage metadata repository environment.
Metadata Catalog and Data Integration (DI) Team
Metadata Catalog and DI Team focuses on defining and managing the content required for the Metadata and Metrics Catalogs. Also, this includes providing the requisite integration of the metadata information as well as metadata maintained by the IT team. The integration is critical in facilitating impact and root cause analysis requirements. Additionally, this team defines the user interface, navigation, and organization of the information contained within the Metadata Catalog to meet the reporting and metrics needs of the company
Database Administrators (DBAs) Team
This team is responsible for providing the needed access to the user community. Also this team handles day to day data requests. The team builds the data accessibility interface to allow for efficient data self-services. Additionally, the Team provides recommendations to the business units, business analytics and reporting analytics representatives as to the flow of data from the system-of-record to each performance metric’s ultimate destination in order to maintain the integrity of each metric. Such recommendations are provided as part of the design and review process between Analytics and IT.
Data Rationalization Services Team
As part of the Data Request Process, the team provides services related to the rationalization of incoming requests for new metrics and/or related data to reduce if not avoid redundancy as well as facilitate the justification and prioritization process in order to ensure that metrics developed are “Metrics That Matter”.
Rationalization services leverage the metadata repository information to determine if the requested metric is similar to existing metric managed by the Analytics Team. Moreover, such services provided information to facilitate the identification of systems of record for the requested metric and includes analysis of each metric candidate source system, granularity, and availability of information required for the requested metric.
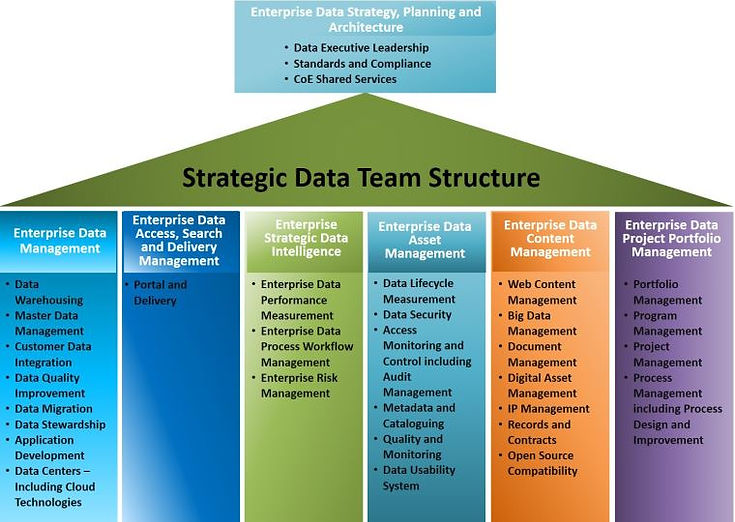
To ensure functionality in the Strategic Data Management, the team can be broken into the following groups:
-
Enterprise Data Strategy, Planning and Architecture
-
Enterprise Data Management
-
Enterprise Access, Search and Delivery Management
-
Enterprise Strategic Data Intelligence
-
Enterprise Data Asset Management
-
Enterprise Data Content Management
-
Enterprise Data Project Portfolio Management
The model focuses on the Strategic Data Management Team’s key operational, administrative, support activities and processes plus the external facing processes required for the effective operation of the Strategic Data Management Team.
The primary deliverables in the model include:
-
Data Governance Processes and Standards
The Strategic Data Management Team works with appropriate stakeholders to define the governance process, standards of operation and procedures. The team has the ongoing role of maintaining the reporting governance standards and processes it implements.
-
Data Management Self Service
Drive and support the deployment of data solutions and associated standards and methodology that support Self Service Data Services. The data solution methodology which is developed and maintained by the Strategic Data Management Team is updated to incorporate enhancements and improvements
Strategic Data Management Team’s customers or/and will have to be trained in how to execute a data self-service activities using the solution.
Data Operating Model

-
Define User Groups and Suite of Tools
The Data Solution contains a suite of tools for customers to use for their data needs. The Strategic Data Management Team classifies users into groups based on data requirements, skill level or other criteria. New or modified user groups are introduced as needed.
-
Define Data Security Roles
The Strategic Data Management Team uses role-based security, which means roles are defined and then access to systems is set up for that role. Subsequent users are added to the predefined roles. This allows for fewer security set ups than security done at the individual level and means less security maintenance.
-
Rationalize Data Requests
Data workers and stakeholders work together to rationalize initial data clusters and then, on an ongoing basis as new data requests are received, they determine if data in the existing catalog and data interface can meet the requestors' needs or if a new data needs have to be created.
-
Coordinate the Design and Development of Data Training Materials
As more data services are added, team members develop new skills that can be of value to meet the customers’ expectation. This service allows for the formalization of the knowledge in the form of intellectual property and provides for its transfer through training, workshops, and other knowledge transfer activities.
-
Data Solution and Related Services
The Data Solution add, remove or enhance components of the solution as the business changes, keeping the solution flexible and responsive to customer needs.
-
Design, Develop and Produce Non-Self-Service Data Requests
Standard data deliverables are also available that are not used as often, or by as many customers - Just one-off request!
-
Data Solution Standards and Techniques
The Strategic Data Management Team has primary responsibility for maintaining the data solution standards and techniques. The Strategic Data Management Team monitors relevant standards and plans for their adoption and integration into the data solutions as they evolve. The Strategic Data Management Team continually assess the efficacy of data solution techniques, adding, updating and improving them as required.
-
Coordinate Data End User Training
The Strategic Data Management Team remains a source of knowledge and experience for the reporting tool suite; therefore, the Strategic Data Management Team works with corporate training to provide guidance on practical and advanced tool usage to be included in Strategic Data Management Team customer self-service training.
-
Future Enhancements
The Strategic Data Management Team keep abreast of data and information delivery tool offerings in the industry. New functionality and enhancements are evaluated for possible benefits to company. The Strategic Data Management Team monitors changes to tools within the data solution, so that these new versions can be evaluated for their functionality and effect.
-
Ongoing Project Support
The Strategic Data Management Team provides project support related to data and information delivery.
Post Implementation
Managing Blocks for the Second Value Proposition include:
The second type of value proposition is that once these capabilities are planned, built or acquired and implemented, these capabilities have to be leveraged correctly to generate returns on investment of the capital invested on data, analytics and business intelligence tools, technologies, infrastructures and manpower. Also, this value proposition implies explicitly and implicitly enhancement of functional benefits, productivity, revenue and profitability otherwise, the investments are waste of scarce resources that could have been deployed somewhere else in the company.
Some of the post implementation of the first value proposition include:
-
Driving Predictive Analytics and Performance Metrics
-
Leveraging Analytics Best Practices to Measure the Impact on Business Results
-
Leveraging the enterprise data through the application of sophisticated analytics methods, tools and processes to provide insights into strategic planning, strategic implementation, monitoring and reporting
-
Ensuring performance management, applifying metrics sensors and driving timely corrective actionable plan
-
Leveraging Command Center deliverables
-
Institutionalizing operational reporting, dashboards and scorecards on the performance of the organization

Driving Predictive Analytics and Performance Metrics for Profitability: Use advanced statistical analysis and modelling including predictive statistical methods to identify the factors that can drive up business-line profitability
Establish data processes that can efficiently be leveraged to provide the needed input for different stakeholders whose responsibilities are different areas of the company that include:
-
Portfolio management
-
Pricing
-
Reserving
-
Sales and Marketing
-
Distribution channels or logistics
-
Customers and Client Management
-
Underwriting
-
Capacity Management
-
Volatility Management
-
Enterprise risk management
-
Strategic Risk
-
Operational Risk
-
Premium Risk
-
Underwriting Risks
-
Marketing Risks
-
Asset/Liability Matching Risks
-
Underwriting Risks (Pricing)
-
Reserving Risks
-
Credit Risks
-
Market Risks
-
Interest Rate Risks
-
Exchange Risks
-
Liquidity Risks
-
-
Capital Management - (Allocation, Economic, Regulatory and Cost of Capital)
Leveraging Analytics Best Practices to Measure the Impact on Business Results:
Use different types of analytics best practices to align with business strategies and also use analytics to measure impact on business results in the areas below:
-
Monthly Reviews and Action Plan
-
Quarterly Reviews and Action Plan
-
Annual Strategic Planning
-
Account Review (Transaction)
-
Growth Playbook
-
Annual Portfolio Review (Annual Portfolio Risk Reports)
-
Capital Management (Economic Capital, Capital Allocation and Capital Requirements)
-
Loss Severity Forecasting
-
Different types of benchmarking analytics that included:
-
Comparative Benchmarking (Comparing the company for internal Improvement)
-
Strategic benchmarking, Process benchmarking, Operational benchmarking and Risk Management benchmarking
-
-
Competitive Benchmarking (Benchmarking to seek Competitive edge or advantages)
-
Risk to Strategic Plan benchmarking, Risk to Rating benchmarking, Product Performance benchmarking and Market Performance benchmarking
-
-
Leveraging the enterprise data through the application of sophisticated analytics methods, tools and processes to provide insights into strategic planning, strategic implementation, monitoring and reporting:
-
Provide input into strategic planning, refining and enriching decision making processes.
-
Customer Buying Behavior:
-
Quantitative predictive analysis of the drivers, barriers, location and dynamics of customer buying behavior so as to generate predictive and prescriptive recommendations to improve marketing processes, products distinction, services uniqueness and marketing effectiveness
-
-
User Experience
-
Customer Analytics provides the clients with deeper insights into their existing or prospective buyers and how best to retain them
-
-
Segmentation
-
Quantitative analysis of customer location, buying power and behavior are leveraged to generate segmentation grid and allows for probability of movement from one grid to the other iand ncluding when best to position the company to benefit from the movement in the grid
-
-
Market Demand Analysis
-
Qualitative and quantitative analysis of what the consumer wants or need and how best to position the company to benefit from the changing demand
-
Ensuring performance management, applifying metrics sensors and driving timely corrective actionable plan:
-
Performance Metrics (PMs)
-
Identify, refine and monitor core performance metrics or “measures that matter” in alignment with the progress towards the achievement of organizational goals.
-
Provides both short and long-term targets to ensure a focus on continuous and breakthrough improvement.
-
-
Key Performance Indicators (KPIs)
-
Provide metric system for estimating and evaluating the level of achievement of quality targets in order to drive actions and decisions.
-
Ensure that current and future-oriented measures align to key operational principles and goals.
-
Develop series of indicators which will be exact, precise, easy to interpret and sensitive to significant changes.
-
Create indicators that identify the gap between performance and expectations with in-built mechanisms to ensure intervention and improvement.
-
-
Sensors
-
Develop and maintain integrated and algorithm based combination of Performance Metrics that allow or automate interventions to better manage operational workflow.
-
-
Timely Corrective Actionable Plan
-
Use different types of analytics best practices to update reports about practices, trends and changes in the company portfolios and communicated the information in business friendly ways to the corporate, business units and functional areas. Many of these reports can be used in different degree as input into decision-making processes that accelerated and motivated timely corrective action plan to meet the expectations of internal stakeholders that included the Board, the leadership and external stakeholders that included the Rating Agencies and the Regulators
-
Analyze the enterprise data to understand the patterns, trend and “what next” to:
Create environment that allows for objective identification and measurement of drivers that can be influenced if there is the need to change the current course of action(s) through Command Centers:
Command Center Deliverables
-
Revenue and Workflow
-
Dynamically focus on meeting revenue goals during the operating hours
-
Dynamically re-distribute work to available resources to meet revenue goals during the operating hours
-
Align employee skill-set with the need of the business horizontal
-
Leverage standardized business processes across the Company for supporting revenue performance
-
-
Productivity, Workflow and Workforce Management
-
Dynamically re-distribute work to available resources
-
Drive process and operational performance monitoring
-
Cross-trained personnel – Skills catalog and defined roles to enhance gap in personnel involvement
-
Ensure continuous process improvement and optimization
-
Drive for standardized business processes across the Company
-
-
Predictive Analytics and Performance Metrics
-
Leverage predictive interventions real-time sensors, alerts and business intelligence to drive Command Center Rapid Response Activities
-
Leverage dynamic data quality management to design and manage measures that matter – Productivity, Conversion Rate, PTP, RPC etc.
-
Manage hierarchy of metrics data from executive/strategic down to employees
-
Connect clients' priorities to Command Center priorities
-
Use advanced statistical analysis and modeling including predictive statistical methods to identify the factors to ensure business-line profitability
-
Create structures and processes that allow for objective identification and measurement of drivers that can be influenced if there is the need to change the current course of action(s) through Operational Reporting, Dashboards and Scorecards:
Operational Reporting, Dashboards and Scorecards on the performance of the organization
-
Provide the Executives with report cards and scorecards on the performance of the organization. Design, develop and increase the use of dashboard across company to:
-
Enhance tactical view for advanced analytics with drill-down capabilities.
-
Display operational data and metrics in near real time.
-
Enhance the company capabilities to monitor enterprise sensors with alert system to ensure quick turnaround on major incidents with issue resolution.
-
-
Monthly Operations Reports (MOR)
-
Provide Monthly review of operations performance
-

Institutionalize Enterprise Risk Management (ERM) Deliverables
Leverage the enterprise data through the application of sophisticated quantitative analytic methods to provide insights that management can use to proactively manage critical business risk issues.
-
Use risk modeling with the empirical properties of exposure related time series such as defaults, recoveries, transitions, and yield spreads of the customers to identify profitable customers. Identified service factors to gain repeat business and referrals. Assess credit worthiness of customers through the use of statistical methods
-
Deliver robust ERM infrastructures and frameworks that have the capabilities for managing risk exposures based on the effectiveness of Board and leadership involvement in all facets of ERM structures and processes
-
Develop reporting process that emphasizes and encourages accountability. The reporting process need to integrate all various data and reports from the business units, functional areas and the corporate to facilitate decision-making that accelerate and motivate timely corrective action plan when needed
-
Set in motion a company-wide understanding of risk policies and risk rules that clearly state the company risk appetite, risk limits and tolerance levels for the violation of the policies and limits in all company areas that include all the SBUs and all the functional areas. Some of these policies are in the areas of:
-
Liquidity risk warning light
-
Credit limit
-
Interest rate limit
-
Counterparty limit
-
Exchange limit
-
Derivative transactions
-
Asset allocations
-
Limits on highly risky products
-
Limits on type of investments
-
Limits on underwriting price fluctuation
-
Limits on Underwriters' approval authority
-
Compliance driven limits
-
-
Establish processes that can efficiently identify and quantify wide varieties of risks in:
-
Portfolio
-
Pricing
-
Reserving
-
Markets
-
Distribution channels or logistics
-
Customers
-
Underwriting
-
Capacity Management
-
Volatility Management
-
-
Emphasize and enhance ERM structures and processes that promote aggregate risk management and efficient risk capital allocation.
-
Determine capital allocation
-
Assess the trends in capital consumption
-
Determine the level of capital required
-
Assess and determine how to derive value from operating capital
-
-
Clearly state the path of how the potential company's risk activities that threaten the risk tolerance should be handled
-
Using scenario analysis, deliver estimated impact on company asset liquidity of triggering events with consequences
-
Institutionalization on fast turnaround time for risk escalation process that prevent risk surprises
-
-
Provide measurement structures and processes to manage ERM to enhance the company's capital growth and stability potential
-
Develop the process layers that can best help to report the ERM structures, processes and activities to meet the expectations of internal stakeholders that include the Board, internal and external stakeholders (including the Rating Agencies). The reporting should include at a minimum:
-
Risk and opportunities
-
Capital utilization and management
-
Financial rating environment
-
Operating plan levers
-
Industry dynamics
-
-
Deliver a Dashboard that integrates performance metrics with risk monitoring and risk metrics reporting that has early warning system
Bringing All Together: Leveraging Data, Analytics and BI as Enablers for A Profitable Company

Leveraging Fast Processing Power For Machine Leaning of the Data, Analytics and BI + In-Memory Processing To Provide Additional Value-added From Analytics
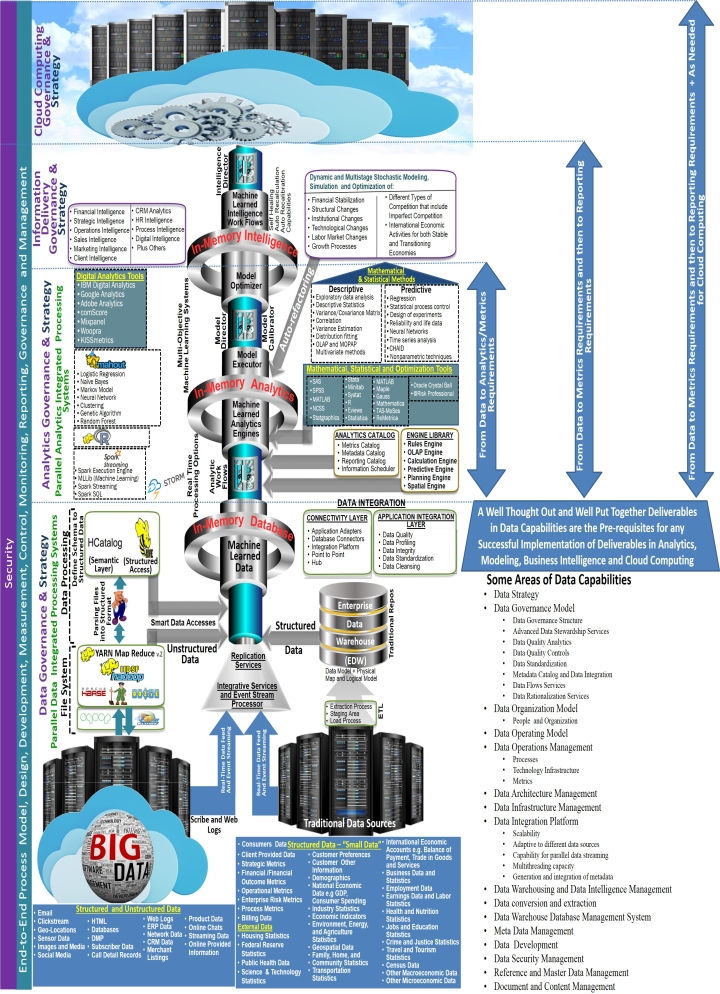